Artificial Intelligence Creates New Possibilities in Radiology
At A Glance
- Artificial intelligence applications in radiology are growing at an astounding pace.
- Artificial intelligence can improve patient diagnosis and speed radiology workflow.
- RSNA can offer peer-reviewed research, images, video and expert commentary to assist in developing news stories on artificial intelligence applications in medicine.
- RSNA Media Relations
1-630-590-7762
media@rsna.org - Linda Brooks
1-630-590-7738
lbrooks@rsna.org - Dionna Arnold
1-630-590-7791
darnold@rsna.org
(April 5, 2018) – The medical use of artificial intelligence (AI) applications, including machine learning and deep learning, is growing at an astounding rate. Applications of the technology in research, radiology workflow and education are increasing exponentially.
The Radiological Society of North America (RSNA) recognizes the value this technology brings to diagnosis and treatment of patients and has launched several research and education initiatives surrounding AI in radiology.
There was a huge interest at AI-related events at RSNA 2017, and there will continue to be a focus on the topic at the upcoming RSNA annual meeting—including an abundance of research, dedicated education areas, and the Machine Learning Showcase on the exhibit floor. In addition, RSNA is cosponsoring a National Institute of Biomedical Imaging and Bioengineering (NIBIB) invitational consensus workshop on artificial intelligence in medical imaging to be held in late summer 2018, and is launching a new online journal, Radiology: Artificial Intelligence, in 2019.
"Radiologists will play a key role in developing AI and making sure the final products meet our needs and the needs of our patients and referring clinicians," said Elliot K. Fishman, M.D., professor of radiology and oncology at the Johns Hopkins University School of Medicine in Baltimore, Md.
While there has been a lot of hype, and even trepidation, about the role AI will ultimately play in radiology, the reality is that AI technologies will add value to the specialty in a number of ways.
"Deep learning is not going to replace us," said Paul Chang, M.D., of the University of the Chicago School of Medicine, during an RSNA 2017 session. "But it will redefine us."
Radiology will need this technology more than ever due to the increasing demands on clinical imaging. Data sets are getting more complex, and there is an increasing need to correlate images with other clinical information in order to implement practices such as radiogenomics.
"Deep learning will help us to meet these new imaging challenges," Dr. Chang said.
Signs of the feverish activity in the AI-radiology arena are everywhere. For example, 2017 saw FDA approval of a self-teaching artificial neural network to help physicians diagnose heart problems and software that provides AI-assisted stroke detection for CT scans and automatically notifies neurovascular specialists by text message. A new smartphone app uses algorithms based on millions of stored images to calculate the likelihood that a mole is becoming cancerous.
AI has also made significant contributions in research of serious medical conditions like Alzheimer's disease, breast cancer, tuberculosis, and pulmonary hypertension.
Leading radiology experts suggest that AI will invigorate the profession and make radiologists more vital than ever to patient care.
"One reason radiology has been so successful is that we are constantly changing," Dr. Fishman said. "If anything, deep learning will guarantee our survival."
Feel free to browse the selection of research and education articles on artificial intelligence at the links below and contact the RSNA media relations team for interviews with leading experts on this exciting topic in radiology.
Images are available for media use. Contact RSNA media relations for assistance.
AI Research
RSNA News: "Machine Learning Aids Pulmonary Hypertension"
RSNA Newsroom: "Machine Learning Identifies Breast Lesions Likely to Become Cancer"
RSNA Newsroom: "New Studies Show Brain Impact of Youth Football"
RSNA Newsroom: "Artificial Intelligence May Help Diagnose Tuberculosis in Remote Areas"
RSNA Newsroom: "Artificial Intelligence May Aid in Alzheimer's Diagnosis"
AI Reviews and Commentary
Radiology: Machine Learning Applied to Alzheimer Disease
Radiology: "When Machines Think: Radiology's Next Frontier"
RSNA News: "Machine Learning is the Next Chapter of Radiology, Not the Last"
AI Background
RadioGraphics: "Machine Learning for Medical Imaging"
RadioGraphics: "Deep Learning: A Primer for Radiologists"
RadioGraphics: "Artificial Intelligence in Radiology: Decision Support Systems"
RadioGraphics: "An Artificial Intelligence Program for the Radiologic Diagnosis of Brain Lesions"
Visit the RSNA 2018 website to learn more about AI research, education and exhibits planned for RSNA's 104th Scientific Assembly and Annual Meeting, Nov. 25 – 30 at McCormick Place, Chicago.
Resources:
Video clips
Images (.JPG and .TIF format)
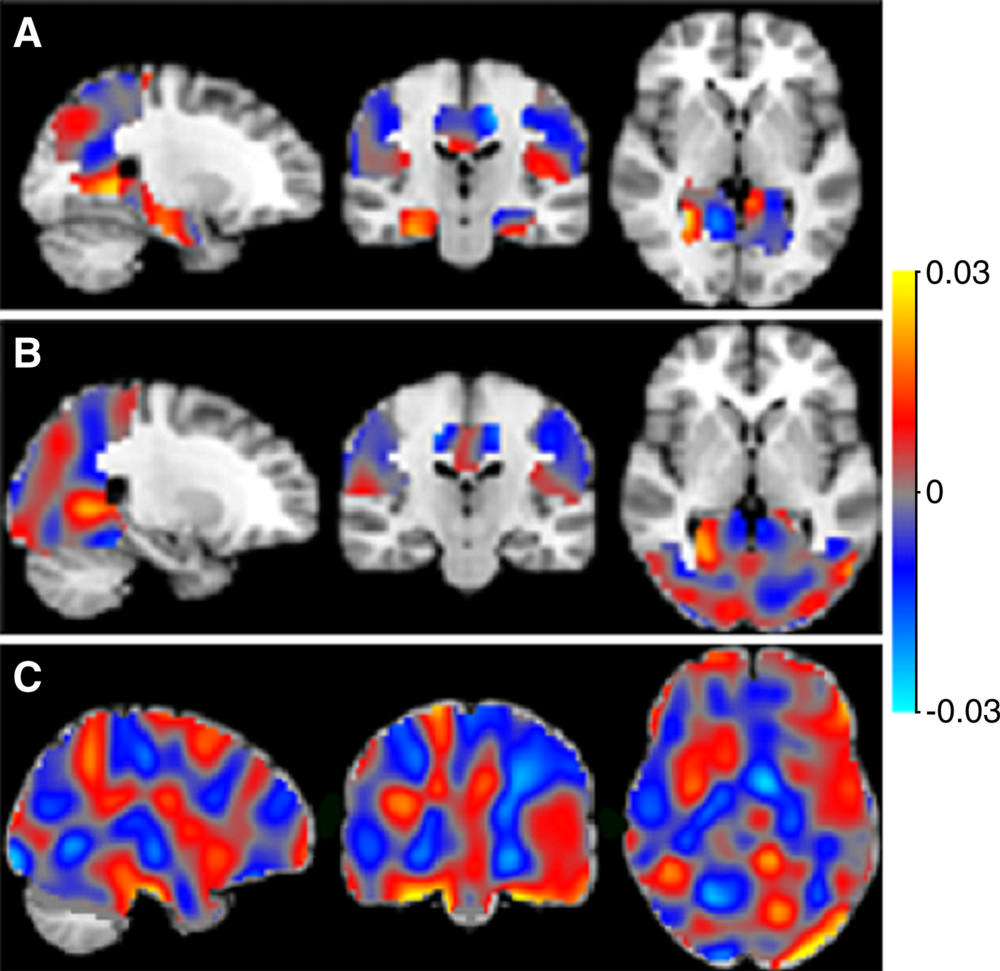
Figure 1. Researchers applied machine learning methods to special type of MRI called arterial spin labeling (ASL) imaging to distinguish different stages of Alzheimer's disease. ASL MRI is used to create images called perfusion maps, which show how much blood is delivered to various regions of the brain. A red color indicates that the intensity at that location contributes to the likelihood of the images belonging to the more advanced stage, and a blue color to the likelihood of belonging to the less advanced stage.
High-res (TIF) version
(Right-click and Save As)
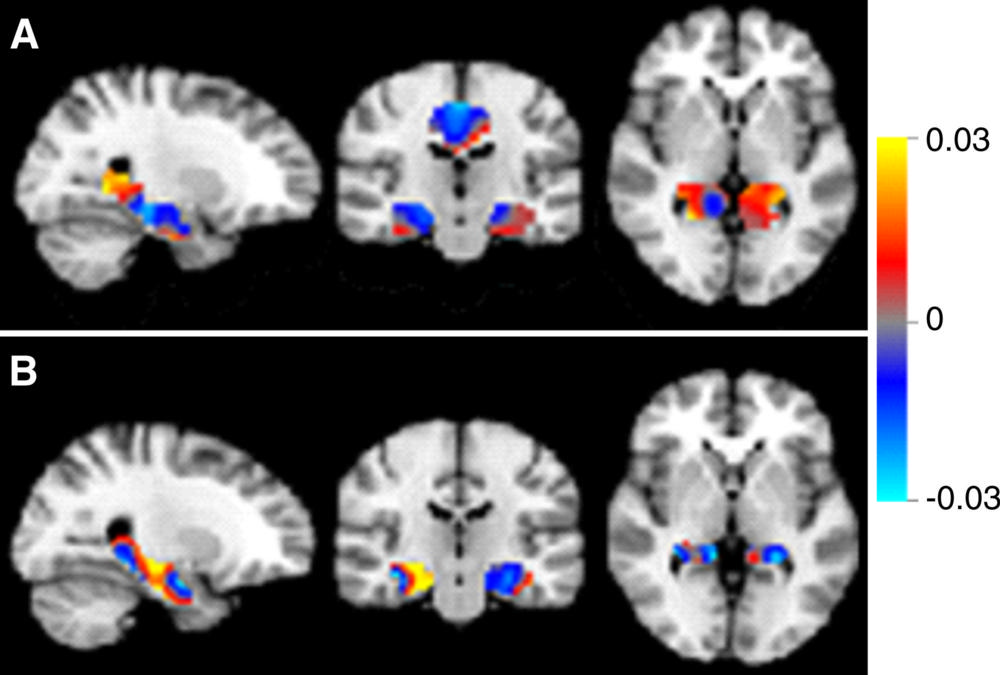
Figure 2. Discrimination maps for classifying mild cognitive impairment (MCI) subgroups, inside the masks that resulted in the highest accuracies. A: between patients with MCI that converted to Alzheimer's disease (MCIc) and patients with subjective cognitive decline; B: between MCIc and patients with MCI that remained stable (MCIs).
High-res (TIF) version
(Right-click and Save As)
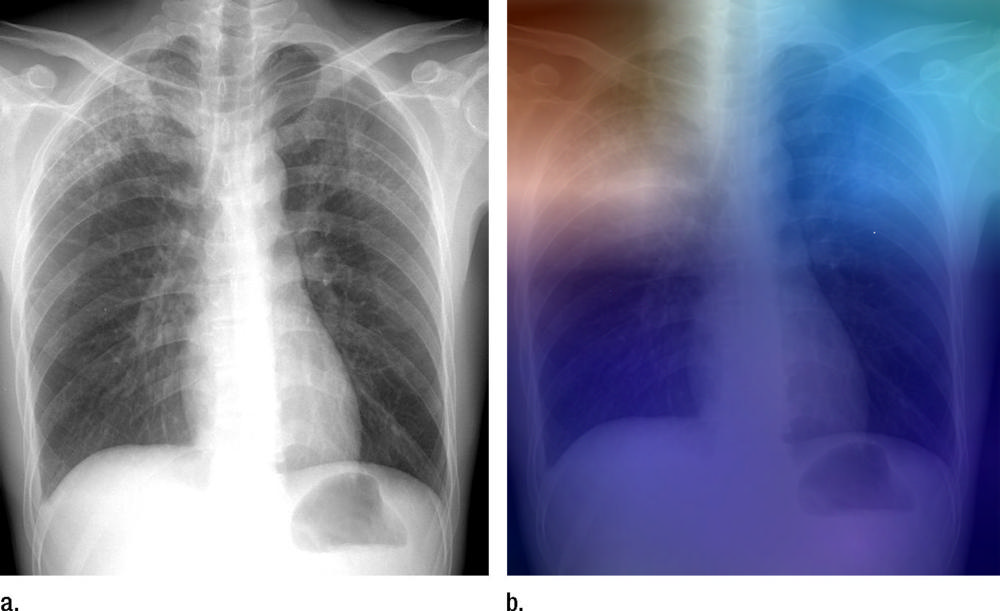
Figure 3. Artificial intelligence models used to identify tuberculosis (TB) on chest X-rays, which may help screening and evaluation efforts in TB-prevalent areas with limited access to radiologists. (a) Posteroanterior chest radiograph shows upper lobe opacities with pathologic analysis–proven active tuberculosis. (b)Same posteroanterior chest radiograph, with a heat map overlay of one of the strongest activations obtained from the fifth convolutional layer after it was passed through the GoogLeNet-TA classifier. The red and light blue regions in the upper lobes represent areas activated by the deep neural network. The dark purple background represents areas that are not activated. This shows that the network is focusing on parts of the image where the disease is present (both upper lobes).
High-res (TIF) version
(Right-click and Save As)
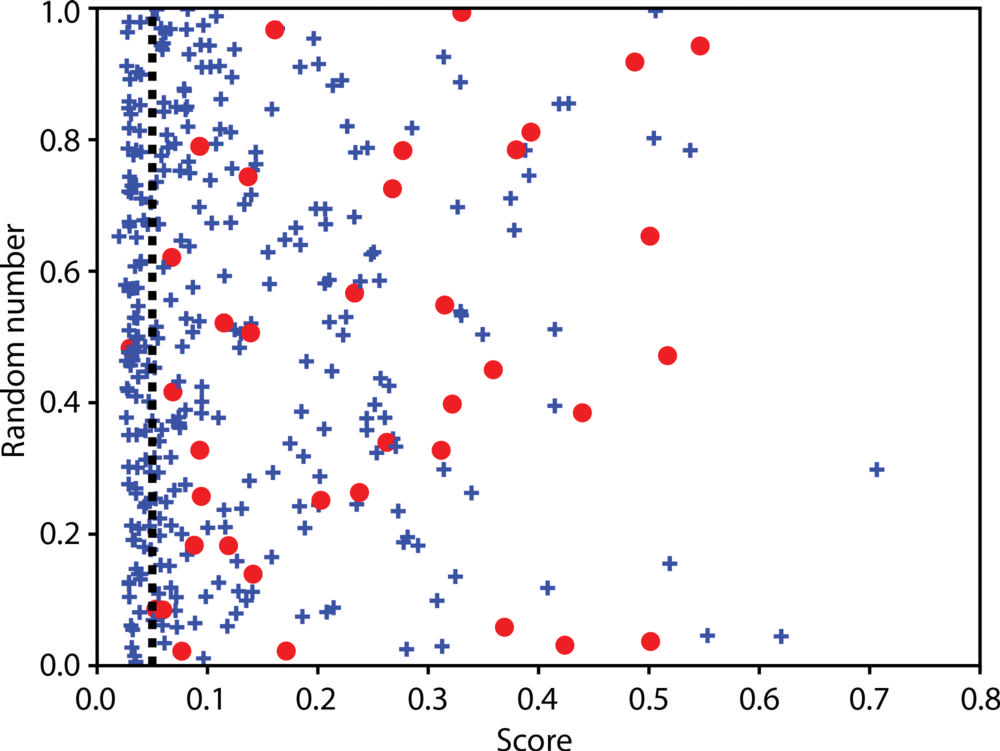
Figure 4. Scatterplot shows score output of machine learning model plotted against a random number in an independent test set of high-risk breast lesions (HRLs). Red circles represent HRLs upgraded to malignancy at surgery, and blue crosses represent HRLs not upgraded to malignancy at surgery. Vertical dotted line indicates 5 percent threshold, below which only one HRL was upgraded to malignancy at surgery.
High-res (TIF) version
(Right-click and Save As)
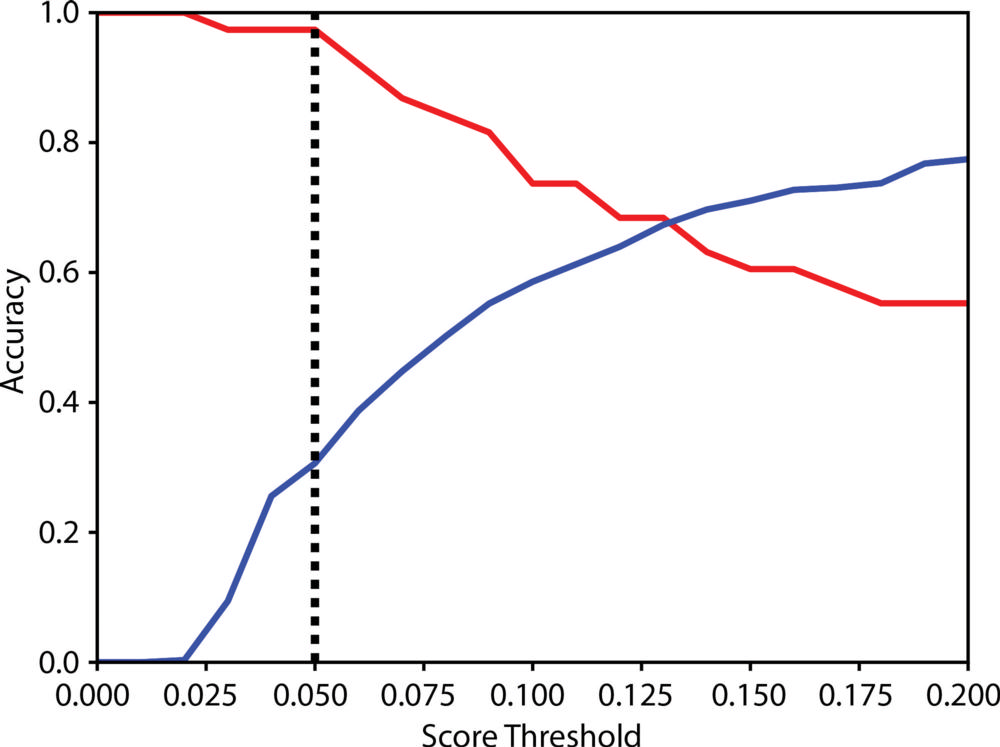
Figure 5. For high-risk breast lesions, graph shows accuracy achieved with machine learning model for independent test set as a function of model output score, both for patients with malignancy (red line) and for patients without malignancy (blue line), in independent test set. Vertical dotted line indicates 5 percent threshold.
High-res (TIF) version
(Right-click and Save As)
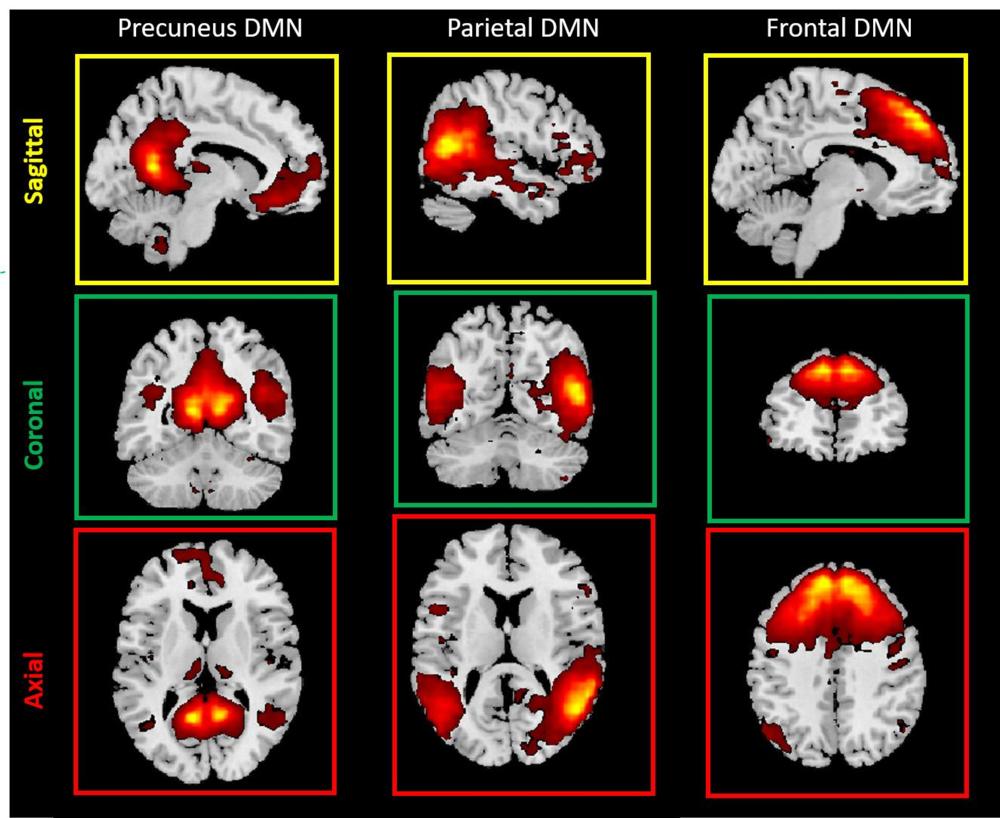
Figure 6. Researchers used machine learning to analyze default mode network subcomponents of the brains of youth football players.
High-res (TIF) version
(Right-click and Save As)