Alzheimer's Disease AI Research Receives RSNA Margulis Award
Released: December 02, 2019
- RSNA Media Relations
1-630-590-7762
media@rsna.org - RSNA 2019 Newsroom
(Dec. 1-5, 2019)
1-312-791-6610 - Linda Brooks
1-630-590-7738
lbrooks@rsna.org - Dionna Arnold
1-630-590-7791
darnold@rsna.org
CHICAGO (Dec. 2, 2019) — The Radiological Society of North America (RSNA) presented its eighth Alexander R. Margulis Award for Scientific Excellence to Jae Ho Sohn, M.D., from the Radiology & Biomedical Imaging Department at the University of California in San Francisco (UCSF), for the article, "A Deep Learning Model to Predict a Diagnosis of Alzheimer Disease by Using 18F-FDG PET of the Brain," published online in November 2018. The groundbreaking study shows the significant potential of artificial intelligence (AI) as a diagnostic tool in Alzheimer's disease (AD). Dr. Sohn was presented with the award today at RSNA 2019 in Chicago.
Named for Alexander R. Margulis, M.D., a distinguished investigator and inspiring visionary in the science of radiology, this annual award recognizes the best original scientific article published in RSNA's peer-reviewed journal Radiology.
A radiology resident with an engineering background, Dr. Sohn co-authored the study with the goal of developing a deep learning algorithm to detect patterns on complex brain images to identify AD as early as possible.
"Alzheimer's is a costly, devastating disease and by the time a clinical diagnosis is made, it's often too late," said Dr. Sohn, who leads the UCSF Big Data in Radiology (BDRAD) multidisciplinary team of physicians and engineers focusing on radiological data science. "We hypothesized we could use AI as an early prediction tool for Alzheimer's in conjunction with other biochemical and imaging tests. If we can detect the disease early enough, physicians have a chance of slowing it down or halting it through therapeutic intervention."
The results far exceeded Dr. Sohn's expectations. Using 18F fluorodeoxyglucose (FDG) PET scans to train a deep learning algorithm, Dr. Sohn and colleagues were able to detect early-stage AD about six years before it was clinically diagnosed.
"This research is groundbreaking," said Radiology editor David A. Bluemke, M.D. "It demonstrates the potential for state-of-the-art AI technology to help interpret the remarkably complex patterns on FDG PET imaging in patients with Alzheimer's disease. Even more hopeful for Alzheimer's patients, this same line of research will also apply to more specific PET tracers for detecting the disease."
The study began when UCSF colleagues Benjamin Franc, M.D., a nuclear medicine specialist, and Youngho Seo, Ph.D., a nuclear medicine physicist, approached Dr. Sohn and undergraduate student Yiming Ding about developing an algorithm to interpret FDG PET brain scans. Research has linked the disease process to changes in metabolism, which can be difficult to recognize. PET scans can measure the uptake of FDG in brain cells, indicating metabolic activity.
The research team used data from the Alzheimer's Disease Neuroimaging Initiative (ADNI), a major multi-site study focused on clinical trials to improve prevention and treatment of this disease. The ADNI dataset included more than 2,100 FDG PET brain images from 1,002 patients. The researchers trained the deep learning algorithm on 90% of the dataset and then tested it on the remaining 10% of the dataset.
Finally, they tested the algorithm on an independent set of 40 FDG PET imaging exams from 40 patients that it had never studied. Results showed that algorithm achieved 82% specificity and 100% sensitivity at detecting the disease an average of more than six years prior to the final diagnosis.
"I was surprised at how high the numbers were, but I already knew AI was going to be more effective than humans," Dr. Sohn said. "The very special nature of AI detects very global, subtle changes that the human eye cannot."
Dr. Sohn, who plans to continue researching the vast capabilities of AI in radiology, was extremely honored to be chosen for Margulis Award, which he believes will open doors for future research.
"I was completely surprised and shocked to receive the award," Dr. Sohn said. "This recognition will pave the way for my research career and will allow me to connect with other researchers doing important work."
Dr. Sohn stresses that further validation with larger and prospective external tests must be performed before the Alzheimer's detection algorithm can be used clinically. However, he believes that researchers united in the fight against Alzheimer's are on the brink of a significant breakthrough.
"I really think we are at an inflection point with Alzheimer's disease," Dr. Sohn said. "I believe we will see major progress in the next decade or two."
Access the study at https://pubs.rsna.org/doi/10.1148/radiol.2018180958.
Note: Copies of RSNA 2019 news releases and electronic images will be available online at RSNA.org/press19 beginning Monday, Dec. 2.
RSNA is an association of over 53,400 radiologists, radiation oncologists, medical physicists and related scientists, promoting excellence in patient care and health care delivery through education, research and technologic innovation. The Society is based in Oak Brook, Ill. (RSNA.org)
Press Resources:
Images (JPG, TIF):
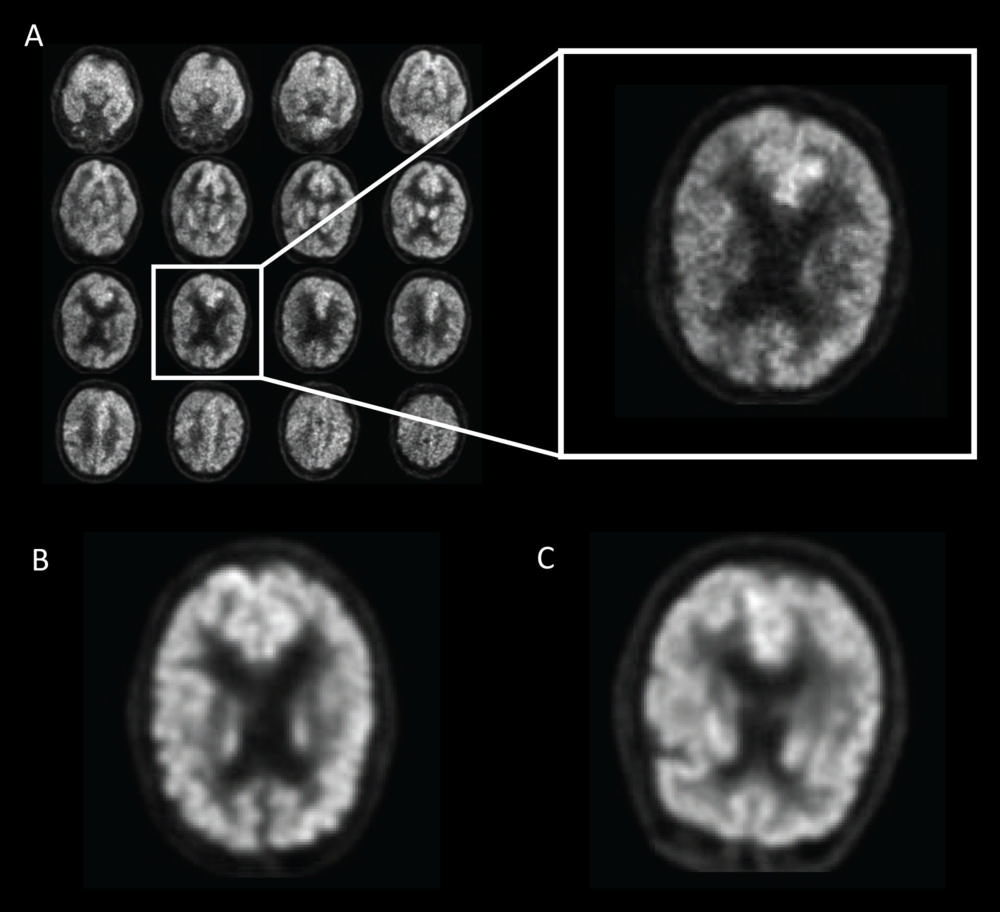
Figure 1. Example of fluorine 18 fluorodeoxyglucose PET images from Alzheimer's Disease Neuroimaging Initiative set preprocessed with the grid method for patients with Alzheimer disease (AD). One representative zoomed-in section was provided for each of three example patients: A, 76-year-old man with AD, B, 83-year-old woman with mild cognitive impairment (MCI), and, C, 80-year-old man with non-AD/MCI. In this example, the patient with AD presented slightly less gray matter than did the patient with non-AD/MCI. The difference between the patient with MCI and the patient with non-AD/MCI appeared minimal to the naked eyes.
High-res (TIF) version
(Right-click and Save As)
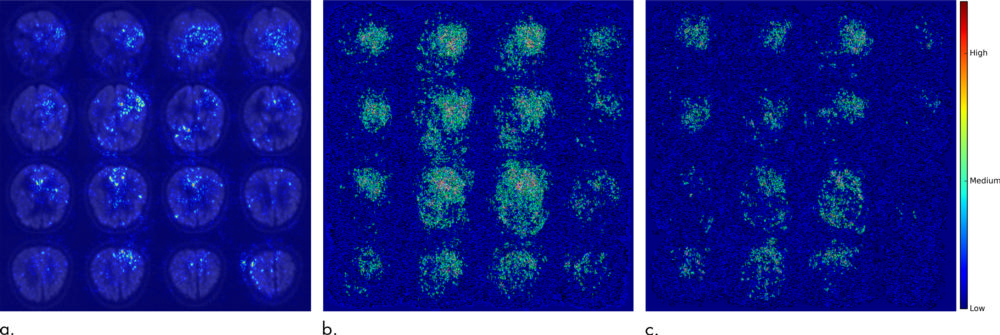
Figure 2. Saliency map of deep learning model Inception V3 on the classification of Alzheimer disease. (a) A representative saliency map with anatomic overlay in 77-year-old man. (b) Average saliency map over 10 percent of Alzheimer's Disease Neuroimaging Initiative set. (c) Average saliency map over independent test set. The closer a pixel color is to the "High" end of the color bar in the image, the more influence it has on the prediction of Alzheimer disease.
High-res (TIF) version
(Right-click and Save As)