Radiologists Share Tips to Prevent AI Bias
Released: May 20, 2025
- RSNA Media Relations
1-630-590-7762
media@rsna.org - Linda Brooks
1-630-590-7738
lbrooks@rsna.org
OAK BROOK, Ill. — Radiologists, computer scientists and informaticists outline pitfalls and best practices to mitigate bias in artificial intelligence (AI) models in an article published today in Radiology, a journal of the Radiological Society of North America (RSNA).
"AI has the potential to revolutionize radiology by improving diagnostic accuracy and access to care," said lead author Paul H. Yi, M.D., associate member (associate professor) in the Department of Radiology and director of Intelligent Imaging Informatics at St. Jude Children's Research Hospital in Memphis, Tennessee. "However, AI algorithms can sometimes exhibit biases, unintentionally disadvantaging certain groups based on age, sex or race."
While there is growing awareness of this issue, there are challenges associated with the evaluation and measurement of algorithmic bias.
In the article, Dr. Yi and colleagues identify key areas where pitfalls occur, as well as best practices and initiatives that should be taken.
"Despite the significant attention this topic receives, there's a notable lack of consensus on key aspects such as statistical definitions of bias, how demographics are categorized, and the clinical criteria used to determine what constitutes a 'significant' bias," Dr. Yi said.
The first such pitfall is the lack of representation in medical imaging datasets. Datasets are essential for the training and evaluation of AI algorithms and can be comprised of hundreds of thousands of images from thousands of patients. Many of the datasets lack demographic information, such as race, ethnicity, age and sex.
For example, in a previous study performed by Dr. Yi and colleagues, of 23 publicly available chest radiograph datasets, only 17% reported race or ethnicity.
To create datasets that are better representations of the wider population, the authors suggest collecting and reporting as many demographic variables as possible, with a suggested minimum set that includes age, sex and/or gender, race and ethnicity. Also, whenever feasible, raw imaging data should be collected and shared without institution-specific post-processing.
The second major issue with bias in AI is the lack of consensus on definitions of demographic groups. This is a challenge because many demographic categories, such as gender or race, are not biological variables but self-identified characteristics that can be informed by society or lived experiences.
The authors note a solution to this would be establishing more specificity with demographic terminologies that better align with societal norms and avoiding combining separate but related demographic categories, such as race and ethnicity or sex and gender.
The final major pitfall is the statistical evaluation of AI biases. At the root of this issue is establishing consensus on the definition of bias, which can have different clinical and technical meanings. In this article, bias is used in the context of demographic fairness and how it reflects differences in metrics between demographic groups.
Once a standard notion of bias is established, the incompatibility of fairness metrics needs to be addressed. Fairness metrics are tools that measure whether a machine learning model treats certain demographic groups differently. The authors stress that there is no universal fairness metric that can be applied to all cases and problems.
The authors suggest using standard and well accepted notions of demographic bias evaluations based on clinically relevant comparisons of AI model performances between demographic groups.
Additionally, they say that it is important to be mindful of the fact that different operating points of a predictive model will result in different performance, leading to potentially different demographic biases. Documentation of these operating points and thresholds should be included in research and by vendors who provide commercial AI products.
According to Dr. Yi, this work provides a roadmap for more consistent practices in measuring and addressing bias. This ensures that AI supports inclusive and equitable care for all people.
"AI offers an incredible opportunity to scale diagnostic capabilities in ways we've never seen before, potentially improving health outcomes for millions of people," he said. "At the same time, if biases are left unchecked, AI could unintentionally worsen healthcare disparities."
"Pitfalls and Best Practices in Evaluation of AI Algorithmic Biases in Radiology." Collaborating with Dr. Yi were Preetham Bachina, B.S., Beepul Bharti, B.S., Sean P. Garin, B.S., Adway Kanhere, M.S.E., Pranav Kulkarni, B.S., David Li, M.D., Vishwa S. Parekh, Ph.D., Samantha M. Santomartino, B.A., Linda Moy, M.D., and Jeremias Sulam, Ph.D.
Radiology is edited by Linda Moy, M.D., New York University, New York, N.Y., and owned and published by the Radiological Society of North America, Inc. (https://pubs.rsna.org/journal/radiology)
RSNA is an association of radiologists, radiation oncologists, medical physicists and related scientists promoting excellence in patient care and health care delivery through education, research and technologic innovation. The Society is based in Oak Brook, Illinois. (RSNA.org)
For patient-friendly information on radiology, visit RadiologyInfo.org.
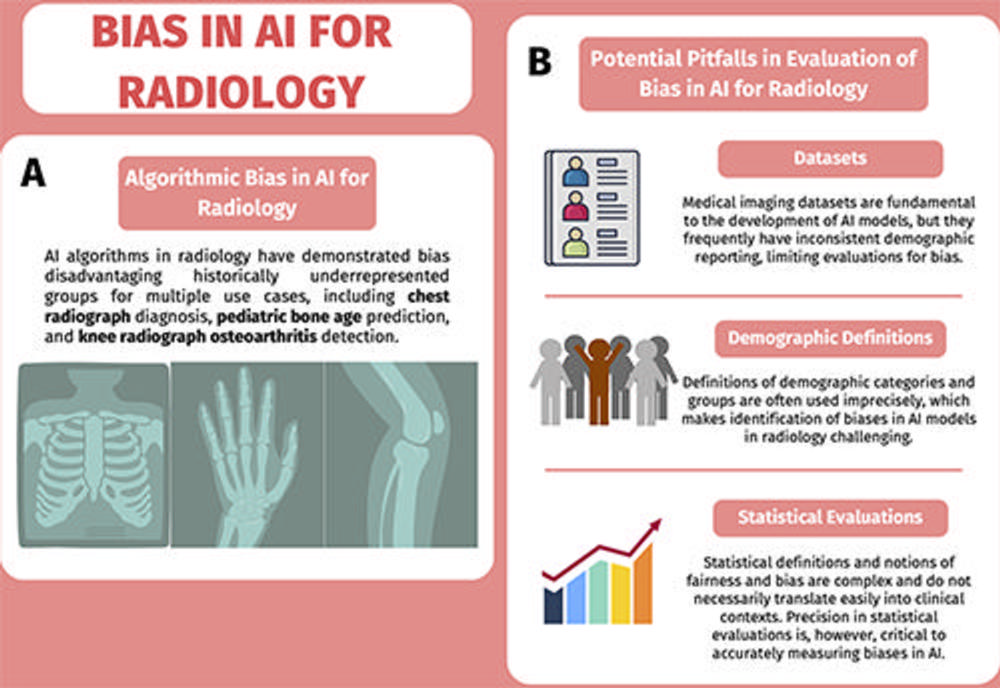
Figure 1. Diagrams of (A) bias and (B) evaluation of bias. (A) Algorithmic bias, or artificial intelligence (AI) bias, in radiology has been demonstrated for multiple use cases. (B) The evaluation of AI bias in radiology has several potential pitfalls related to datasets, demographic definitions, and statistical evaluations. The neural network graphics created by Loxaxs from Wikimedia Commons were modified under Creative Commons license (CC0 1.0).
High-res (TIF) version
(Right-click and Save As)
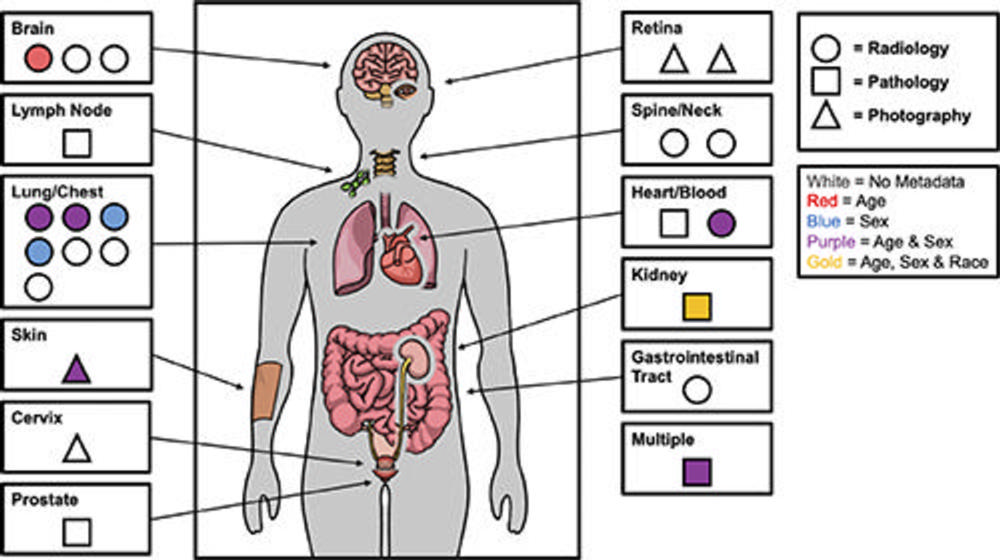
Figure 2. Figure illustrates demographic reporting practices of medical imaging datasets on Kaggle platform (https://www.kaggle.com) stratified by body part, imaging type, and types of demographic metadata reported. The majority of datasets did not report any demographics and those that did, reported age and/or sex only with the exception of one that reported age, sex, and race. Data are from Garin et al. “Medical Imaging Data Science Competitions Should Report Dataset Demographics and Evaluate for Bias.” Image courtesy of Sean P. Garin.
High-res (TIF) version
(Right-click and Save As)
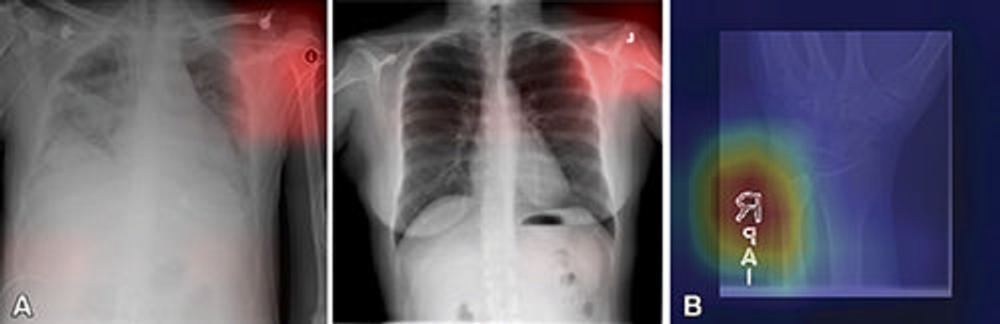
Figure 3. (A) Images from a deep learning (DL) model in radiology that can learn to identify confounding features related to bias and unfair predictions, including laterality markers (image annotations indicate the side of the body being viewed [right vs left]) to identify the hospital at which a chest X-ray was obtained. Images adapted and reprinted from Zech, et al. “Variable Generalization Performance of a Deep Learning Model to Detect Pneumonia in Chest Radiographs: A Cross-sectional Study,” an open-source article, published under the Creative Commons license (CC BY 4.0). (B) Image from a DL model that can make a diagnosis of radiographic abnormality on extremity radiographs, also known as shortcut learning. Reprinted, with permission, from Yi, et al. “Deep Learning Algorithms for Interpretation of Upper Extremity Radiographs: Laterality and Technologist Initial Labels as Confounding Factors.”
High-res (TIF) version
(Right-click and Save As)
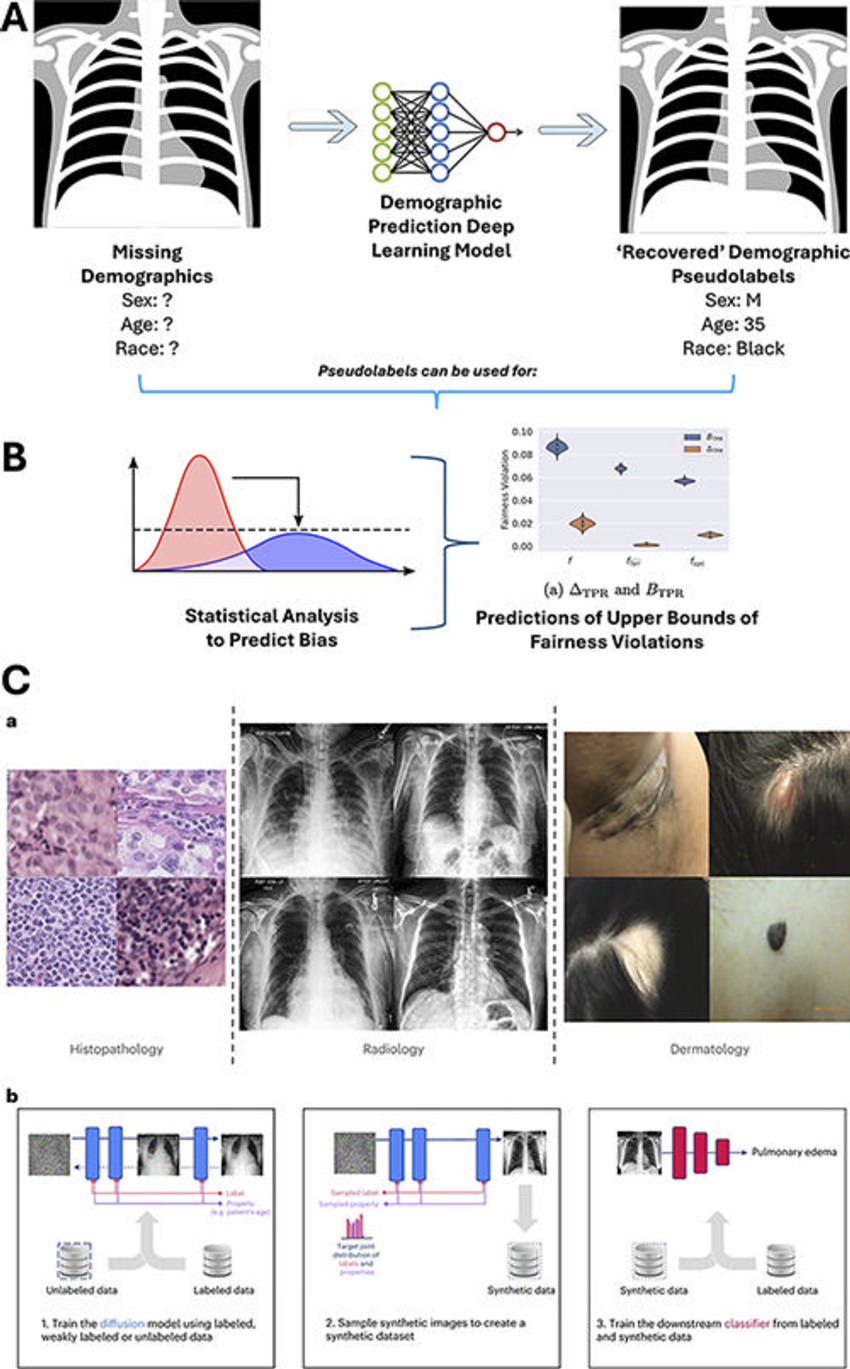
Figure 4. Technical approaches to addressing pitfalls in dataset limitations for the evaluation of artificial intelligence (AI) bias in radiology. (A) Deep learning models trained to identify patient-reported demographics on medical images can be used to “recover” pseudolabels for demographics, which allow for estimates of dataset diversity and potential biases. The neural network graphic (middle graphic) was modified under Creative Commons license (CC0 1.0) and the chest radiograph graphics (right and left graphics) were created by Jmarchn from Wikimedia Commons, under Creative Commons license (CC BYSA 3.0). (B) These pseudolabels (labels assigned to unlabeled data from the outputs of AI algorithms trained to predict that label) can be used in conjunction with advanced statistical methods to predict upper bounds for the degree of fairness violations and performance disparities for an AI model tested on a dataset even in the absence of demographic labels. Graph on the left is a free image from Rawpixel, licensed under a Creative Commons license (CC0 1.0) (https://www.rawpixel.com/). Chart on the right is adapted and reprinted from Bharti, et al. “Estimating and Controlling for Fairness via Sensitive Attribute Predictors,” an open-source article, published under the Creative Commons license (CC BY 4.0). B = worst-case fairness violation of TPR, TPR = true-positive rate. (C) Generative AI models (AI trained to generate new data, including images, text, and video) can be used to create synthetic medical images to augment datasets, which can be used to train subsequent disease classification AI models that have decreased fairness disparities. Reprinted from Ktena I, Wiles O, Albuquerque I, et al. “Generative Models Improve Fairness of Medical Classifiers Under Distribution Shifts,” an open-access article, published under Creative Commons license (CC BY 4.0)
High-res (TIF) version
(Right-click and Save As)
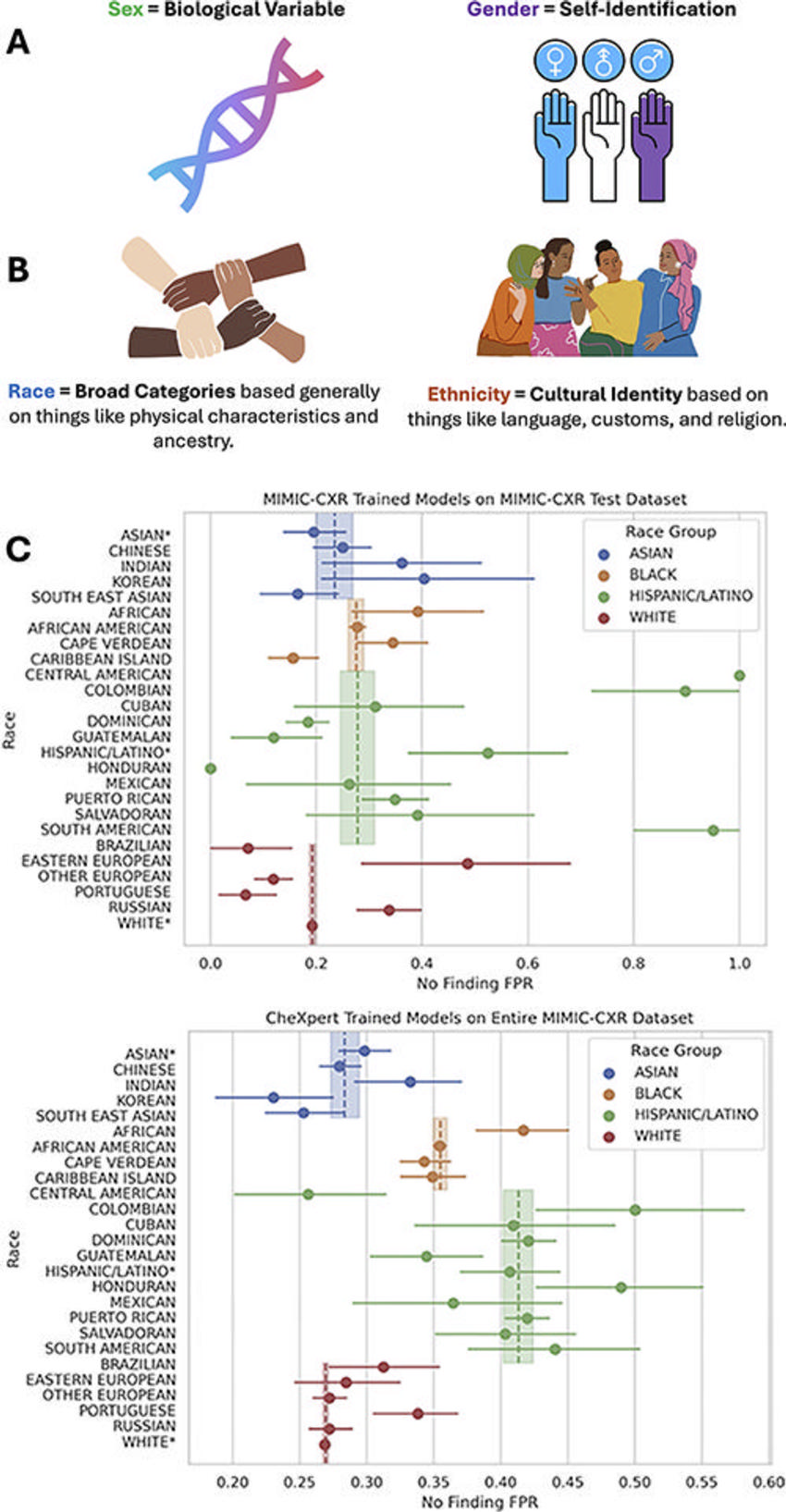
Figure 5. Demographic definitions are often used imprecisely but have specific semantic distinctions and meanings that are critical for the evaluation of artificial intelligence (AI) bias in radiology. (A) Male, female, and other related categories can fall under sex and/or gender, which are two separate categories. (B) Similarly, race and ethnicity are often conflated, but represent two distinct concepts and categories. Using granular ethnicity labels (eg, Korean or Indian) can help identify clinically meaningful performance disparities in AI models in radiology that can go hidden when measuring such biases using coarse race labels (eg, Asian). (C) Forest plots show granular underdiagnosis rates. In this example, there are several hidden underdiagnosis disparities identified within each coarse racial group when evaluating granular ethnicity labels that often exceed the variation between coarse racial groups. Granular groups labeled with an asterisk are the patients who only reported a coarse race or ethnicity. FPR = false-positive rate.
High-res (TIF) version
(Right-click and Save As)
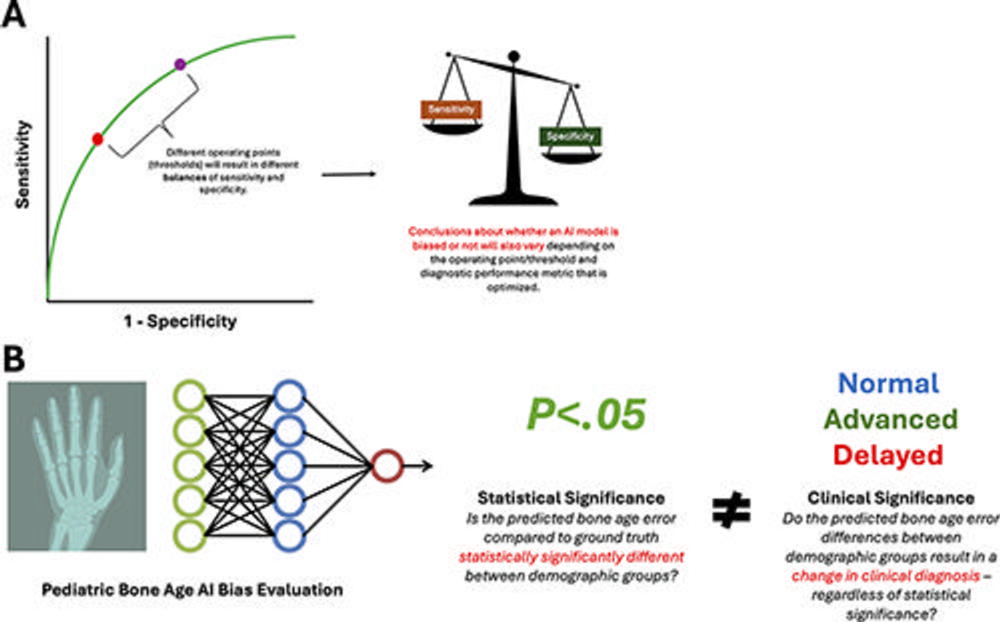
Figure 6. Statistical evaluations of artificial intelligence (AI) bias in radiology have several pitfalls and considerations to ensure clinically relevant conclusions are drawn. These include (A) recognizing the paradox of the incompatibility of fairness metrics, where different fairness metrics cannot be fulfilled simultaneously, analogous to how a receiver operating characteristic curve requires choice of threshold points that have trade-offs between sensitivity and specificity, and (B) distinguishing between statistical and clinical significance when evaluating measured biases. The neural network graphic created by Loxaxs from Wikimedia Commons was modified under Creative Commons license (CC0 1.0).
High-res (TIF) version
(Right-click and Save As)