AI Can Help Rule Out Abnormal Pathology on Chest X-rays
Released: August 20, 2024
- RSNA Media Relations
1-630-590-7762
media@rsna.org - Linda Brooks
1-630-590-7738
lbrooks@rsna.org - Imani Harris
1-630-481-1009
iharris@rsna.org
OAK BROOK, Ill. — A commercial artificial intelligence (AI) tool used off-label was effective at excluding pathology and had equal or lower rates of critical misses on chest X-ray than radiologists, according to a study published today in Radiology, a journal of the Radiological Society of North America (RSNA).
Recent developments in AI have sparked a growing interest in computer-assisted diagnosis, partly motivated by the increasing workload faced by radiology departments, the global shortage of radiologists and the potential for burnout in the field. Radiology practices have a high volume of unremarkable (no clinically significant findings) chest X-rays, and AI could possibly improve workflow by providing an automatic report.
Researchers in Denmark set out to estimate the proportion of unremarkable chest X-rays where AI could correctly exclude pathology without increasing diagnostic errors. The study included radiology reports and data from 1,961 patients (median age, 72 years; 993 female), with one chest X-ray per patient, obtained from four Danish hospitals.
“Our group and others have previously shown that AI tools are capable of excluding pathology in chest X-rays with high confidence and thereby provide an autonomous normal report without a human in-the-loop,” said lead author Louis Lind Plesner, M.D., from the Department of Radiology at Herlev and Gentofte Hospital in Copenhagen, Denmark. “Such AI algorithms miss very few abnormal chest radiographs. However, before our current study, we didn’t know what the appropriate threshold was for these models.”
The research team wanted to know whether the quality of mistakes made by AI and radiologists was different and if AI mistakes, on average, are objectively worse than human mistakes.
The AI tool was adapted to generate a chest X-ray “remarkableness” probability, which was used to calculate specificity (a measure of a medical test’s ability to correctly identify people who do not have a disease) at different AI sensitivities.
Two chest radiologists, who were blinded to the AI output, labeled the chest X-rays as “remarkable” or “unremarkable” based on predefined unremarkable findings. Chest X-rays with missed findings by AI and/or the radiology report were graded by one chest radiologist—blinded to whether the mistake was made by AI or radiologist—as critical, clinically significant or clinically insignificant.
The reference standard labeled 1,231 of 1,961 chest X-rays (62.8%) as remarkable and 730 of 1,961 (37.2%) as unremarkable. The AI tool correctly excluded pathology in 24.5% to 52.7% of unremarkable chest X-rays at greater than or equal to 98% sensitivity, with lower rates of critical misses than found in the radiology reports associated with the images.
Dr. Plesner notes that the mistakes made by AI were, on average, more clinically severe for the patient than mistakes made by radiologists.
“This is likely because radiologists interpret findings based on the clinical scenario, which AI does not,” he said. “Therefore, when AI is intended to provide an automated normal report, it has to be more sensitive than the radiologist to avoid decreasing standard of care during implementation. This finding is also generally interesting in this era of AI capabilities covering multiple high-stakes environments not only limited to health care.”
AI could autonomously report more than half of all normal chest X-rays, according to Dr. Plesner. “In our hospital-based study population, this meant that more than 20% of all chest X-rays could have been potentially autonomously reported using this methodology, while keeping a lower rate of clinically relevant errors than the current standard,” he said
Dr. Plesner noted that a prospective implementation of the model using one of the thresholds suggested in the study is needed before widespread deployment can be recommended.
“Using AI to Identify Unremarkable Chest Radiographs for Automatic Reporting.” Collaborating with Dr. Plesner were Felix C. Müller, M.D., Ph.D., Mathias W. Brejnebøl, M.D., Christian Hedeager Krag, M.D., Lene C. Laustrup, M.D., Finn Rasmussen, M.D., D.M.Sc., Olav Wendelboe Nielsen, M.D., Ph.D., Mikael Boesen, M.D., Ph.D., and Michael B. Andersen, M.D., Ph.D.
Radiology is edited by Linda Moy, M.D., New York University, New York, N.Y., and owned and published by the Radiological Society of North America, Inc. (https://pubs.rsna.org/journal/radiology)
RSNA is an association of radiologists, radiation oncologists, medical physicists and related scientists promoting excellence in patient care and health care delivery through education, research and technologic innovation. The Society is based in Oak Brook, Illinois. (RSNA.org)
For patient-friendly information on chest X-rays, visit RadiologyInfo.org.
Images (JPG, TIF):
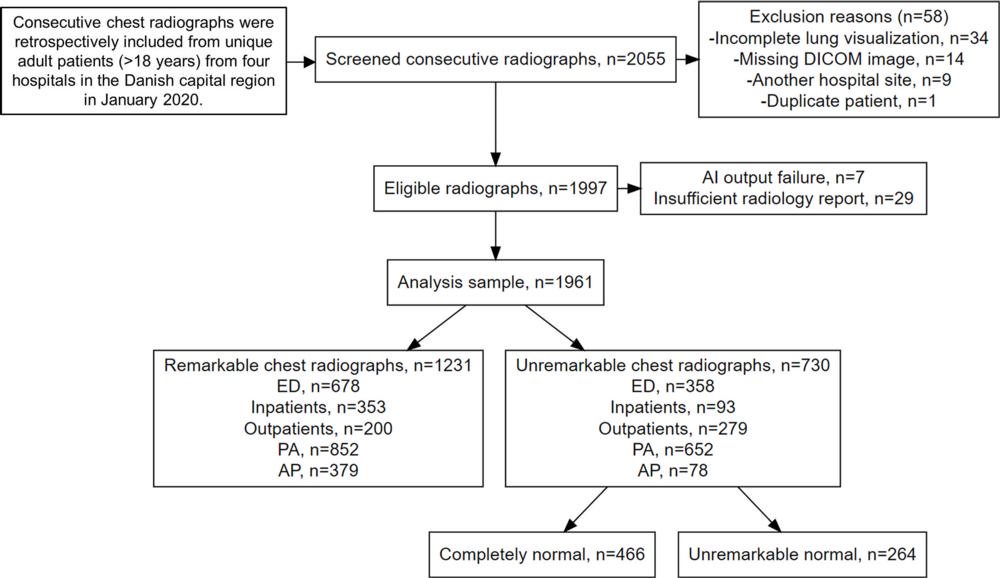
Figure 1. Flowchart shows the inclusion process. A remarkable chest X-ray was defined as having one or more abnormal findings. A completely normal chest X-ray was defined as having no abnormal findings. An unremarkable normal chest X-ray was defined as normal but with one or more unremarkable findings. AI = artificial intelligence, AP = anteroposterior, DICOM = Digital Imaging and Communications in Medicine, ED = emergency department, PA = posteroanterior
High-res (TIF) version
(Right-click and Save As)
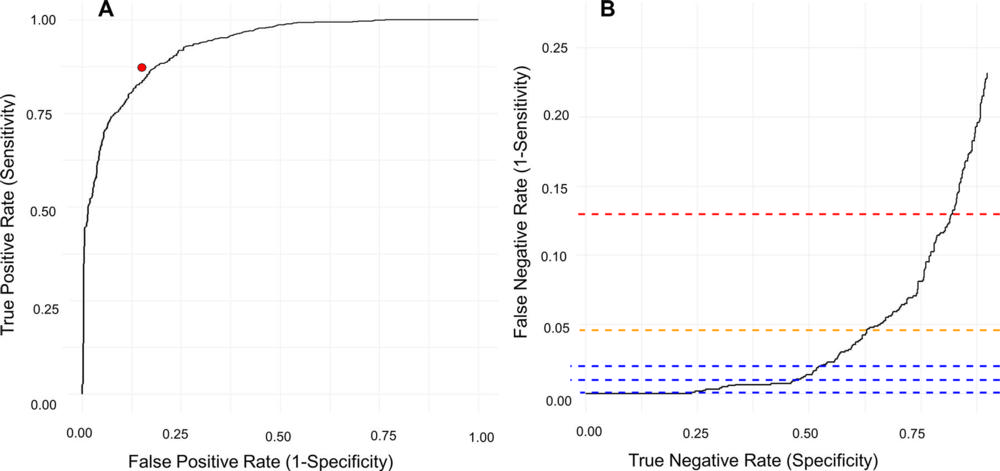
Figure 2. Diagnostic performance of the artificial intelligence (AI) tool for the classification of remarkable and unremarkable chest X-rays. (A) Receiver operating characteristic curve shows the performance of the pooled radiologists writing the clinical radiology reports (red dot). (B) Inverted receiver operating characteristic curve shows only the high sensitivity area. The curve has been inverted to illustrate how specificity increases with increasing false-negative rates. The red dashed line indicates the false-negative rate found in the radiology reports; the yellow dashed line indicates the AI threshold producing an equal number of critical missed chest X-rays (1.1%); and the blue dashed lines (from bottom to top) indicate the 99.9%, 99.0%, and 98.0% AI sensitivity thresholds, respectively.
High-res (TIF) version
(Right-click and Save As)
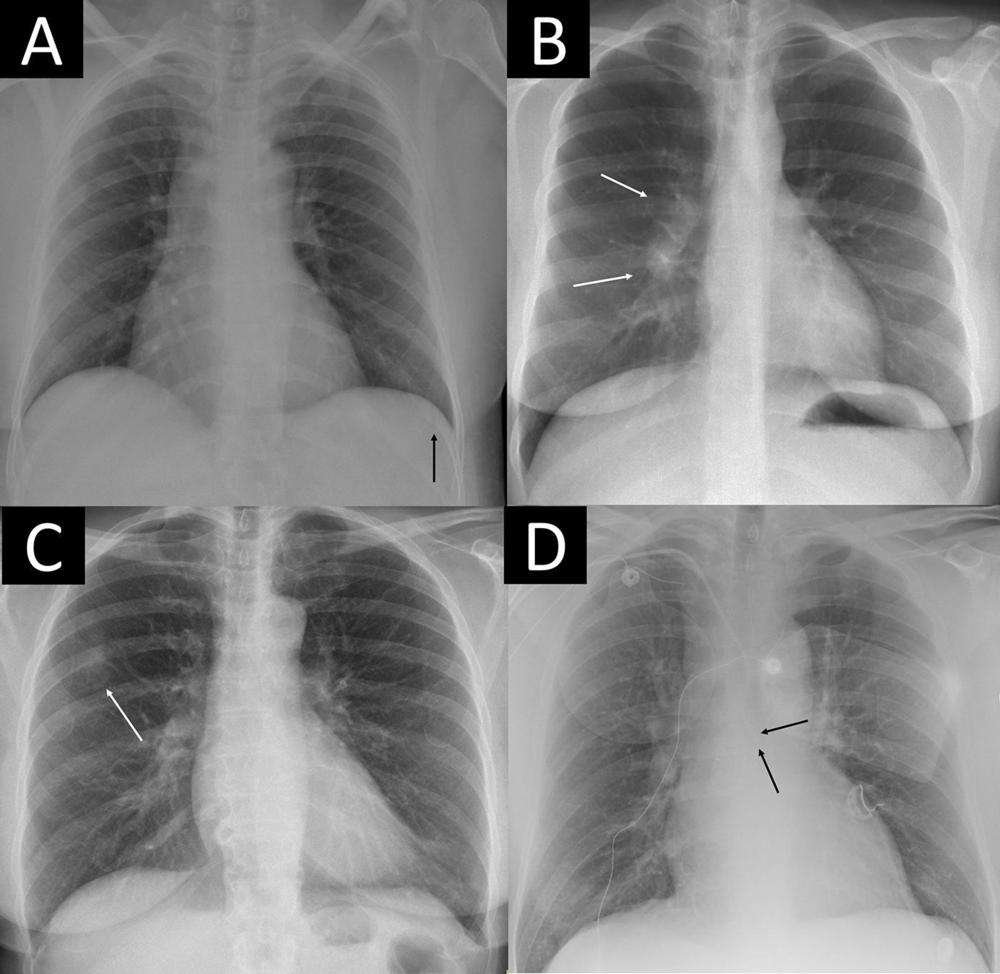
Figure 3. Four examples of remarkable chest X-rays with missed critical findings. The artificial intelligence (AI) tool was postprocessed by the AI vendor by scaling each of the 85 remarkable individual predictions to a normalized value and using the case-level highest of the scaled scores as the overall probability score from 0 to 1 for “remarkableness” (ie, the probability for abnormal or remarkable findings by the AI). (A) Chest X-ray in a 49-year-old female patient shows a slightly visible acute rib fracture (arrow) that was missed by the AI at all thresholds and also missed by the radiology report. (B) Chest X-ray in a 30-year-old female patient shows enlarged hilar lymph nodes (arrows) missed by the radiology report but not the AI at any threshold. (C) Chest X-ray in a 67-year-old female patient shows a tumor mimicking pleural plaque (arrow) that was reported in the radiology report (where the patient was referred for CT) and missed by the AI at the 98.0% threshold but not the 99.0% and 99.9% thresholds. (D) Chest X-ray in a 64-year-old male patient shows a central venous catheter possibly entering the azygos vein (arrows), which was classified as unremarkable in the radiology report. The AI missed the critical finding at the 98.0% threshold but not the 99.0% and 99.9% thresholds.
High-res (TIF) version
(Right-click and Save As)
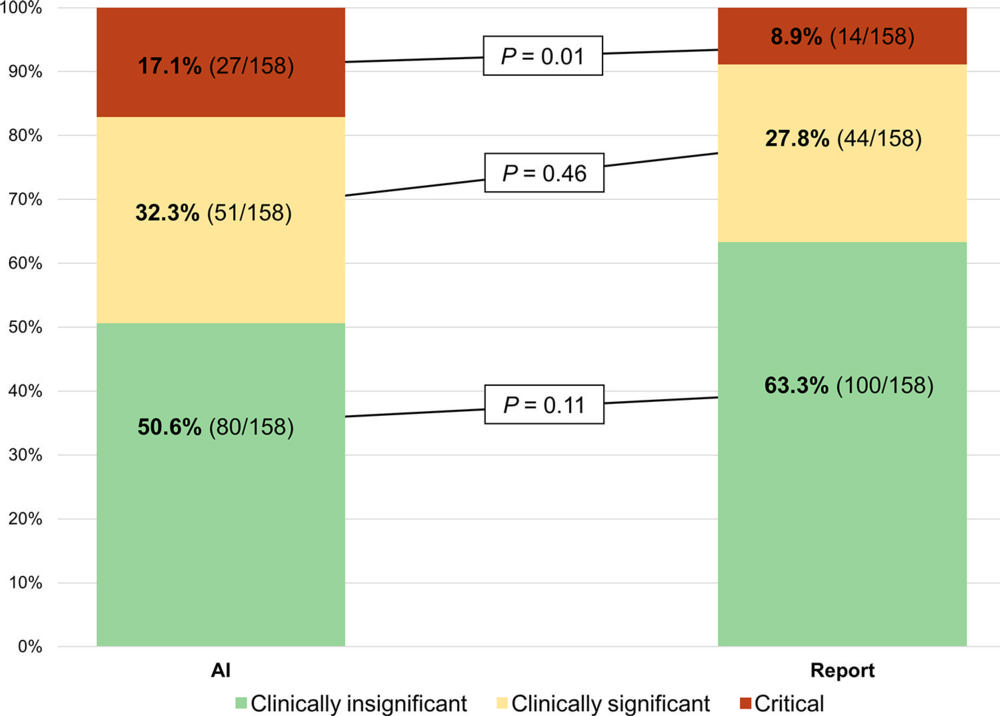
Figure 4. Stacked bar chart shows the estimated clinical consequence of misses by the artificial intelligence (AI) tool at a fixed sensitivity threshold and the radiology reports. There were 158 chest X-rays in which the radiology report classified a remarkable chest X-ray as unremarkable, corresponding to a sensitivity of 87.2%. When fixing the AI threshold to this sensitivity, the AI produced the same number of misses and thereby simulated the radiologist operating point as closely as possible. Clinical consequence was labeled by a thoracic radiologist (M.B.A.) who had full access to previous X-ray history but was blinded to which reader (ie, AI or report) had missed the finding. Missed chest X-rays were classified into three groups based on the following definitions: (a) clinically insignificant (“would put the finding in the report but would not expect any clinical action to be taken from it”), (b) clinically significant (“would put the finding in the report and would expect a clinical action to be taken from it”), and (c) critical (“would immediately call the referring physician and/or check the patient file to see if the finding had already been noticed by the clinician and acted upon, or explicitly state in the report that follow-up imaging is warranted”). P values were obtained using the McNemar test, with continuity correction for each group individually.
High-res (TIF) version
(Right-click and Save As)
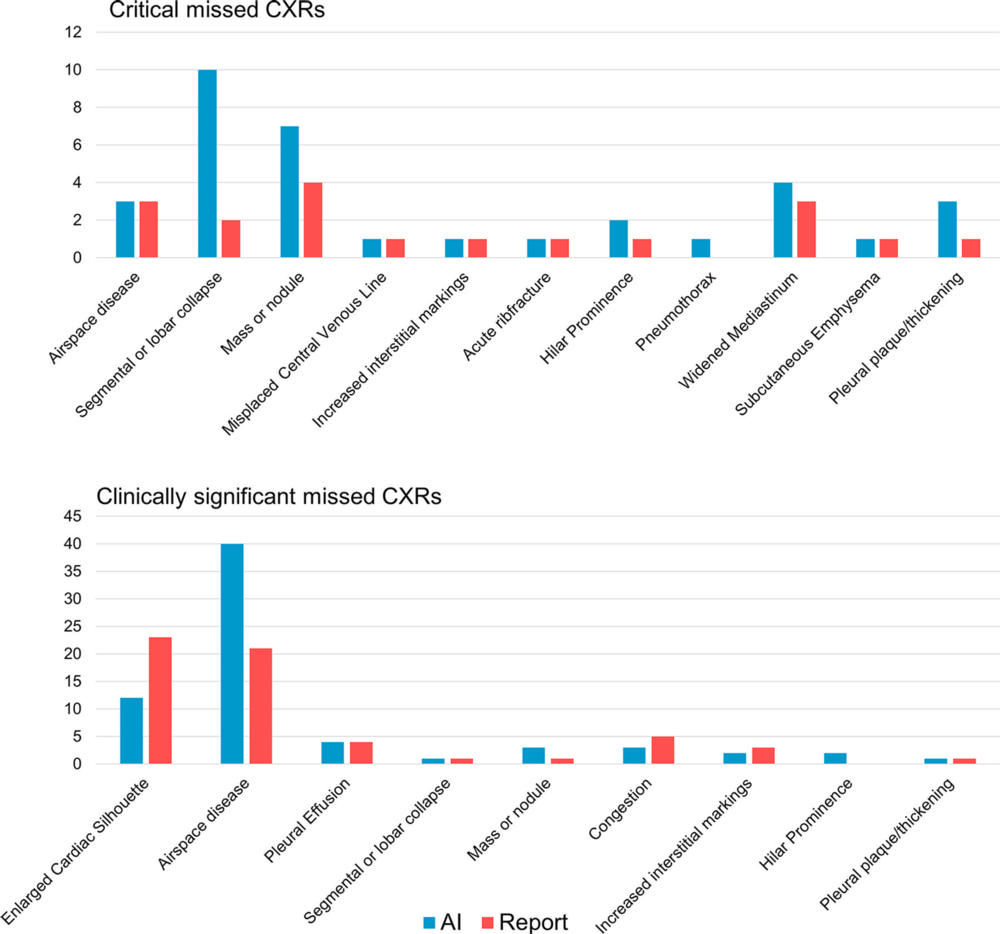
Figure 5. Bar graphs show the numbers of all chest X-ray findings present in remarkable chest X-rays (CXRs) labeled as unremarkable by either the artificial intelligence (AI) tool or radiology reports and classified as critical (top) or clinically significant (bottom). The AI was fixed to a similar sensitivity (87.2%) as the radiology reports. Some findings were missed by both the AI and report, and these were included in both the AI and report columns. The findings were labeled by two radiologists in consensus, with a third radiologist for adjudication, as the reference standard.
High-res (TIF) version
(Right-click and Save As)