AI Model Effective in Detecting Prostate Cancer
Released: August 06, 2024
- RSNA Media Relations
1-630-590-7762
media@rsna.org - Linda Brooks
1-630-590-7738
lbrooks@rsna.org - Imani Harris
1-630-481-1009
iharris@rsna.org
OAK BROOK, Ill. — A deep learning model performs at the level of an abdominal radiologist in the detection of clinically significant prostate cancer on MRI, according to a study published today in Radiology, a journal of the Radiological Society of North America (RSNA). The researchers hope the model can be used as an adjunct to radiologists to improve prostate cancer detection.
Prostate cancer is the second most common cancer in men worldwide. Radiologists typically use a technique that combines different MRI sequences (called multiparametric MRI) to diagnose clinically significant prostate cancer. Results are expressed through the Prostate Imaging-Reporting and Data System version 2.1 (PI-RADS), a standardized interpretation and reporting approach. However, lesion classification using PI-RADS has limitations.
"The interpretation of prostate MRI is difficult," said study senior author Naoki Takahashi, M.D., from the Department of Radiology at the Mayo Clinic in Rochester, Minnesota. "More experienced radiologists tend to have higher diagnostic performance."
Applying artificial intelligence (AI) algorithms to prostate MRI has shown promise for improving cancer detection and reducing observer variability, which is the inconsistency in how people measure or interpret things that can lead to errors. However, a major drawback of existing AI approaches is that the lesion needs to be annotated (adding a note or explanation) by a radiologist or pathologist at the time of initial model development and again during model re-evaluation and retraining after clinical implementation.
"Radiologists annotate suspicious lesions at the time of interpretation, but these annotations are not routinely available, so when researchers develop a deep learning model, they have to redraw the outlines," Dr. Takahashi said. "Additionally, researchers have to correlate imaging findings with the pathology report when preparing the dataset. If multiple lesions are present, it may not always be feasible to correlate lesions on MRI to their corresponding pathology results. Also, this is a time-consuming process."
Dr. Takahashi and colleagues developed a new type of deep learning model to predict the presence of clinically significant prostate cancer without requiring information about lesion location. They compared its performance with that of abdominal radiologists in a large group of patients without known clinically significant prostate cancer who underwent MRI at multiple sites of a single academic institution. The researchers trained a convolutional neural network (CNN)—a sophisticated type of AI that is capable of discerning subtle patterns in images beyond the capabilities of the human eye—to predict clinically significant prostate cancer from multiparametric MRI.
Among 5,735 examinations in 5,215 patients, 1,514 examinations showed clinically significant prostate cancer. On both the internal test set of 400 exams and an external test set of 204 exams, the deep learning model's performance in clinically significant prostate cancer detection was not different from that of experienced abdominal radiologists. A combination of the deep learning model and the radiologist's findings performed better than radiologists alone on both the internal and external test sets.
Since the output from the deep learning model does not include tumor location, the researchers used something called a gradient-weighted class activation map (Grad-CAM) to localize the tumors. The study showed that for true positive examinations, Grad-CAM consistently highlighted the clinically significant prostate cancer lesions.
Dr. Takahashi sees the model as a potential assistant to the radiologist that can help improve diagnostic performance on MRI through increased cancer detection rates with fewer false positives.
"I do not think we can use this model as a standalone diagnostic tool," Dr. Takahashi said. "Instead, the model's prediction can be used as an adjunct in our decision-making process."
The researchers have continued to expand the dataset, which is now twice the number of cases used in the original study. The next step is a prospective study that examines how radiologists interact with the model's prediction.
"We'd like to present the model's output to radiologists and assess how they use it for interpretation and compare the combined performance of radiologist and model to the radiologist alone in predicting clinically significant prostate cancer," Dr. Takahashi said.
"Fully Automated Deep Learning Model to Detect Clinically Significant Prostate Cancer at MRI." Collaborating with Dr. Takahashi were Jason C. Cai, M.D., Hirotsugu Nakai, M.D., Ph.D., Shiba Kuanar, Ph.D., Adam T. Froemming, M.D., Candice W. Bolan, M.D., Akira Kawashima, M.D., Ph.D., Hiroaki Takahashi, M.D., Ph.D., Lance A. Mynderse, M.D., Chandler D. Dora, M.D., Mitchell R. Humphreys, M.D., Panagiotis Korfiatis, Ph.D., Pouria Rouzrokh, M.D., M.P.H., M.H.P.E., Alexander K. Bratt, M.D., Gian Marco Conte, M.D., Ph.D., and Bradley J. Erickson, M.D., Ph.D.
Radiology is edited by Linda Moy, M.D., New York University, New York, N.Y., and owned and published by the Radiological Society of North America, Inc. (https://pubs.rsna.org/journal/radiology)
RSNA is an association of radiologists, radiation oncologists, medical physicists and related scientists promoting excellence in patient care and health care delivery through education, research and technologic innovation. The Society is based in Oak Brook, Illinois. (RSNA.org)
For patient-friendly information on MRI and prostate imaging, visit RadiologyInfo.org.
Video (MP4):
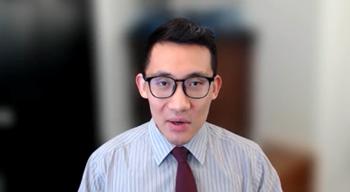
Lead author Jason C. Cai, M.D., discusses his research on fully automated deep learning model to detect clinically significant prostate cancer at MRI.
Download MP4
(Right-click and Save As)
Images (JPG, TIF):
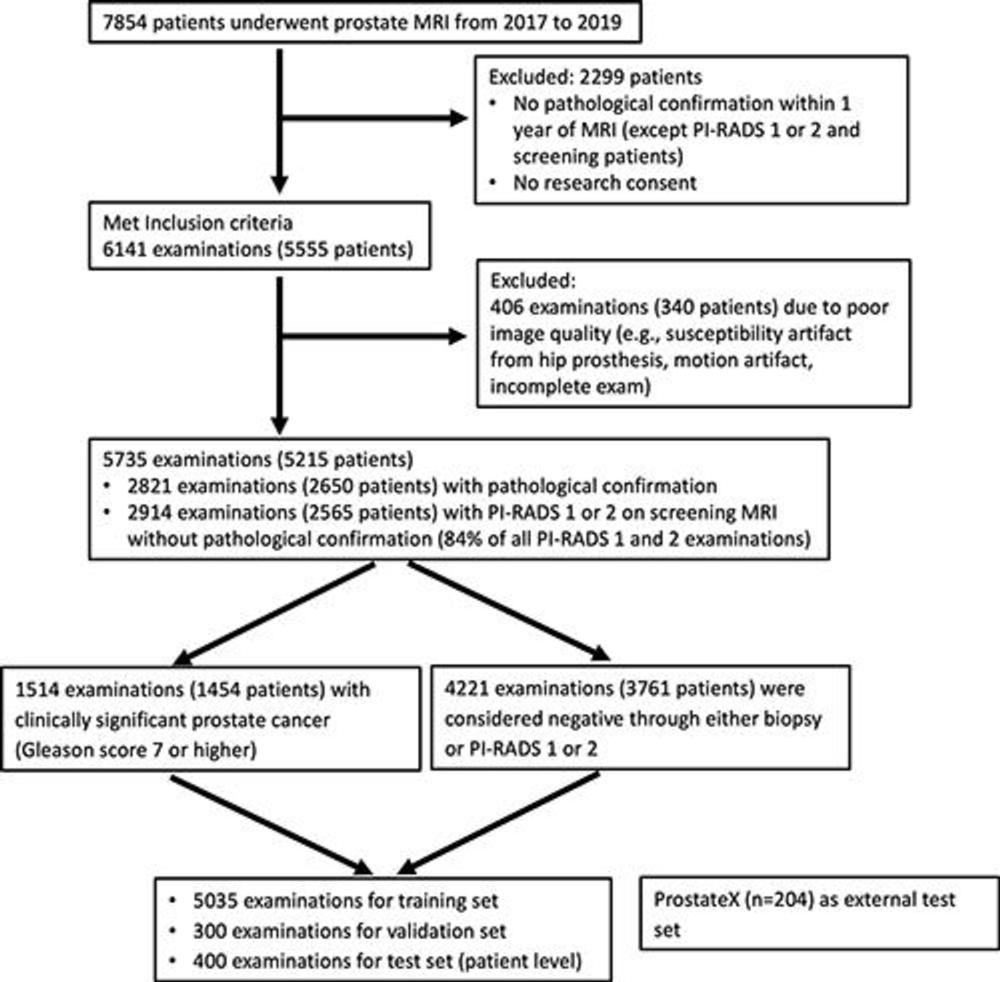
Figure 1. Flowchart shows inclusion and exclusion criteria and patient characteristics. PI-RADS = Prostate Imaging Reporting and Data System.
High-res (TIF) version
(Right-click and Save As)
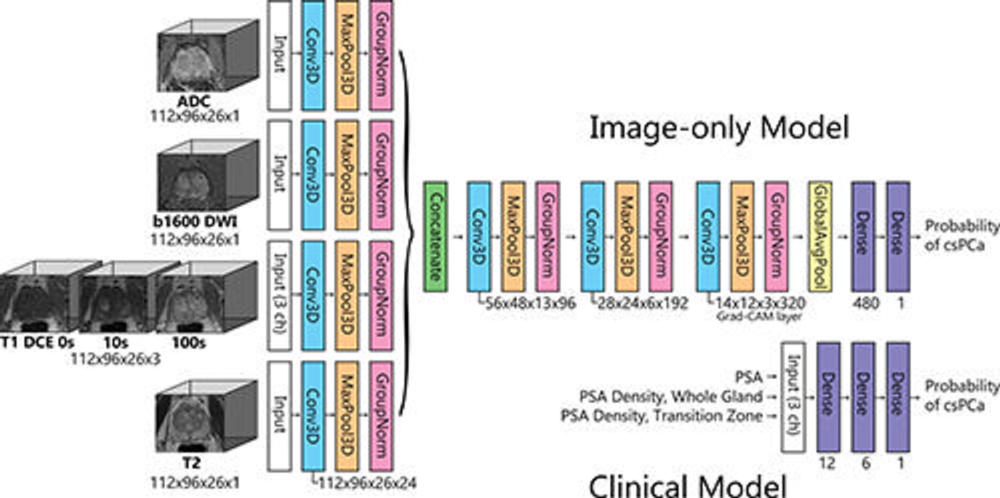
Figure 2. Diagram shows the architecture of the image-only model and clinical model. The image-only model consisted of one set of three-dimensional (3D) convolutions (3D convolutional kernel [Conv3D], maximum pooling [MaxPool3D], and group normalization [GroupNorm]) for each input volume (T2, diffusion-weighted imaging [DWI], apparent diffusion coefficient [ADC], and dynamic contrast-enhanced [DCE]), followed by concatenation, three additional sets of 3D convolutions, global average pooling (GlobalAvgPool), and two fully connected layers. The clinical model consists of a neural network of two fully connected layers with prostate-specific antigen (PSA) level, whole-gland PSA density, and transition zone PSA density as input. The output from each model was the probability of clinically significant prostate cancer (csPCa). ch = channel, Grad-CAM = gradient-weighted class activation map.
High-res (TIF) version
(Right-click and Save As)
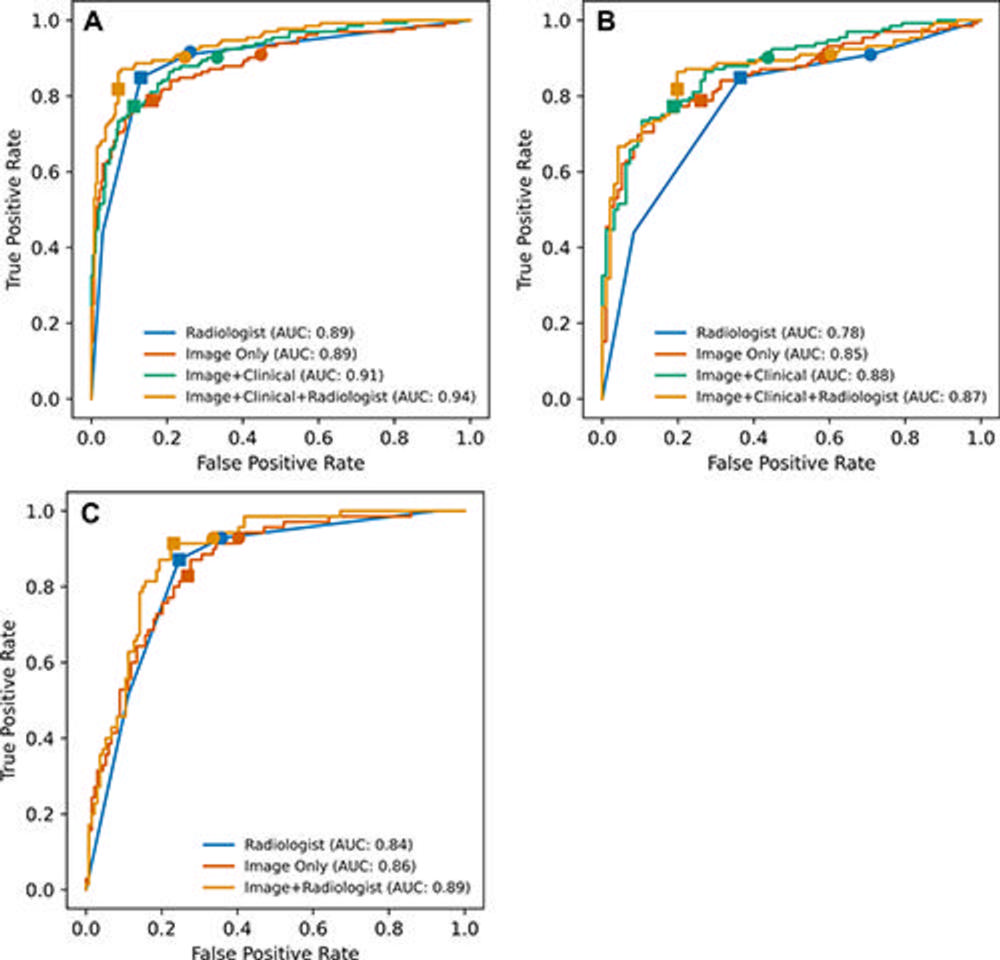
Figure 3. Receiver operating characteristic curves of the models and radiologists in predicting clinically significant prostate cancer. (A) Internal test set, all cases (n = 400). (B) Internal test set, pathology-proven cases only (n = 228), and (C) external (ProstateX) test set (n = 204). Filled squares represent true-positive rate (TPR) and false-positive rate (FPR) at optimal thresholds. Optimal thresholds were determined with use of the Youden J statistic using the validation set, and then the thresholds were applied to the internal and external test sets. Filled circles represent thresholds equivalent to that of radiologists at Prostate Imaging Reporting and Data System 3 (TPR of 91% in the internal test set and TPR of 93% in the external test set). In A, TPR and FPR of the radiologist and image+clinical+radiologist models are identical, but the plots are slightly shifted for better visualization. AUC = area under the receiver operating characteristic curve.
High-res (TIF) version
(Right-click and Save As)
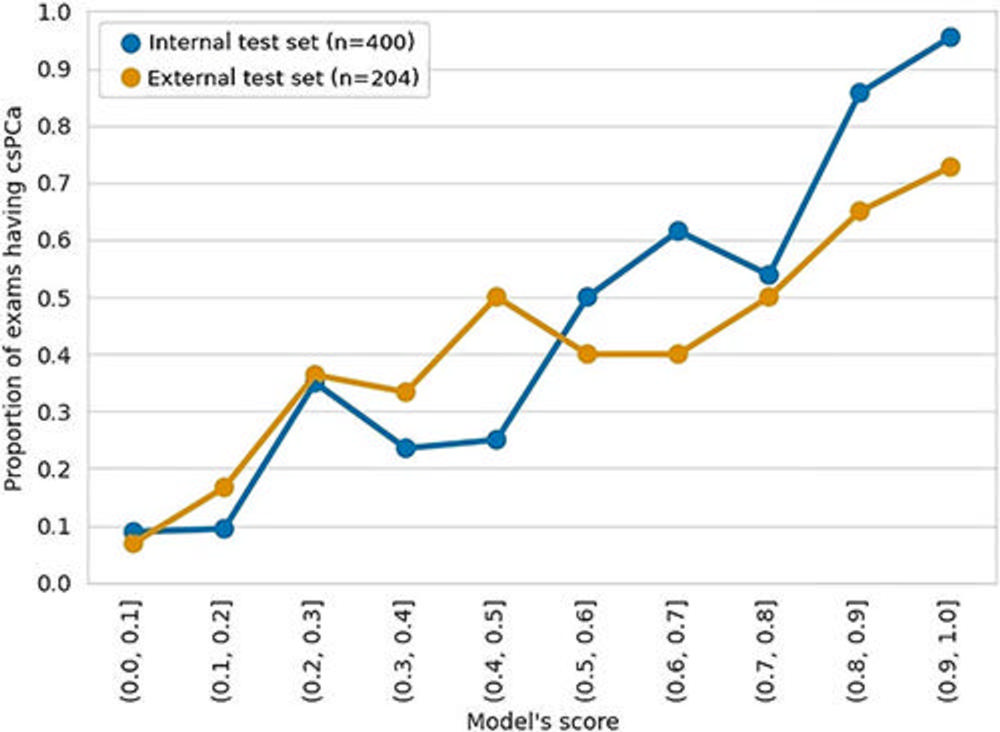
Figure 4. Calibration plot of the image-only model. The output of the image-only model (probability score) was categorized into 10 bins with increments of 0.1, and the proportion of clinically significant prostate cancer (csPCa) was calculated for each bin.
High-res (TIF) version
(Right-click and Save As)
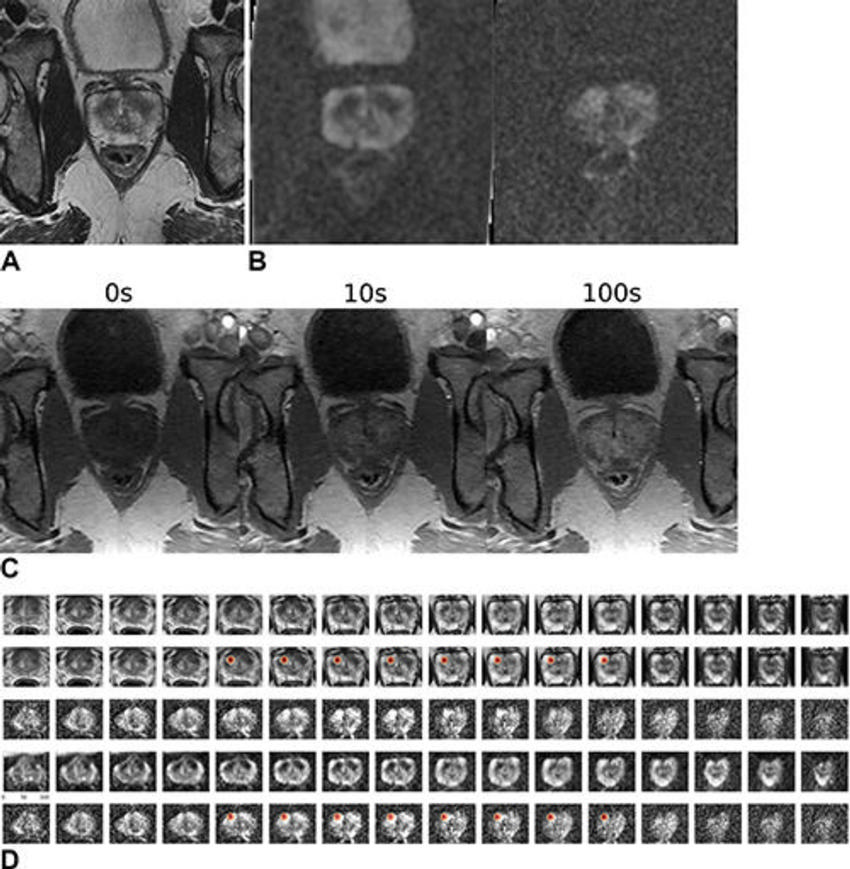
Figure 5. Images in a 59-year-old male patient who underwent MRI for clinical suspicion of prostate cancer (internal test set). The patient subsequently underwent prostatectomy and had a 1.5-cm prostate adenocarcinoma (Gleason score 3 + 4) in the right mid anterior to bilateral posterior inferior prostate gland. The model’s output (patient level probability) was 0.83. Only the lesion in the right lobe was highlighted by the gradient-weighted class activation map (Grad-CAM). The radiologist graded this examination as Prostate Imaging Reporting and Data System (PI-RADS) 4 for the right lobe lesion and PI-RADS 3 for the left lobe lesion. (A) T2-weighted image (representative section). (B) Apparent diffusion coefficient map (representative section, left) and high-b-value diffusion-weighted image (representative section, right). (C) T1 dynamic contrast-enhanced images (representative sections). (D) Volumetric composite of T2-weighted images (rows 1 and 2), diffusion-weighted images (rows 3 and 5), and apparent diffusion coefficient maps (row 4), with superimposed Grad-CAMs (rows 2 and 5). All images are in the transverse plane.
High-res (TIF) version
(Right-click and Save As)
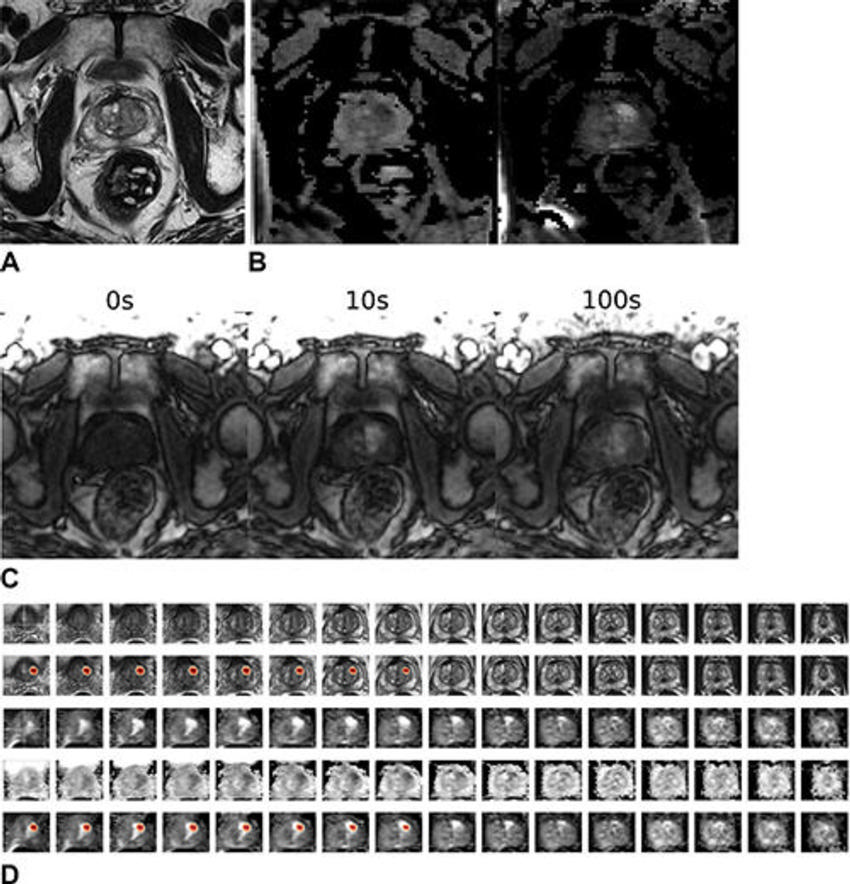
Figure 6. Images in a 64-year-old male patient who underwent MRI for clinical suspicion of prostate cancer (external test set). The patient was subsequently diagnosed with clinically significant prostate cancer in the left anterior transition zone at the base of the prostate gland. The model’s output (patient-level probability) was 0.97. The radiologist graded this examination as Prostate Imaging Reporting and Data System 3 for the left lobe lesion. (A) T2-weighted image (representative section). (B) Apparent diffusion coefficient map (representative section, left) and high-b-value diffusion-weighted image (representative section, right). (C) T1 dynamic contrast-enhanced images (representative sections). (D) Volumetric composite of T2-weighted images (rows 1 and 2), diffusion-weighted images (rows 3 and 5), and apparent diffusion coefficient maps (row 4), with superimposed gradient-weighted class activation maps (rows 2 and 5). All images are in the transverse plane.
High-res (TIF) version
(Right-click and Save As)