Researchers Test Large Language Model that Preserves Patient Privacy
Released: October 10, 2023
At A Glance
- Researchers tested the feasibility of using a locally run large language model (LLM) to label key findings from chest X-ray reports, while preserving patient privacy.
- The LLM was asked to identify and label the presence or absence of 13 specific findings on the chest X-ray reports.
- The LLM’s performance was comparable to the current reference standard.
- RSNA Media Relations
1-630-590-7762
media@rsna.org - Linda Brooks
1-630-590-7738
lbrooks@rsna.org - Imani Harris
1-630-481-1009
iharris@rsna.org
OAK BROOK, Ill. — Locally run large language models (LLMs) may be a feasible option for extracting data from text-based radiology reports while preserving patient privacy, according to a new study from the National Institutes of Health Clinical Center (NIH CC) published in Radiology, a journal of the Radiological Society of North America (RSNA). LLMs are deep-learning models trained to understand and generate text in a human-like way.
Recently released LLM models such as ChatGPT and GPT-4 have garnered attention. However, they are not compatible with healthcare data due to privacy constraints.
“ChatGPT and GPT-4 are proprietary models that require the user to send data to OpenAI sources for processing, which would require de-identifying patient data,” said senior author Ronald M. Summers, M.D., Ph.D., senior investigator in the Radiology and Imaging Sciences Department at the NIH. “Removing all patient health information is labor-intensive and infeasible for large sets of reports.”
In this study, led by Pritam Mukherjee, Ph.D., staff scientist at the NIH CC, researchers tested the feasibility of using a locally run LLM, Vicuna-13B, to label key findings from chest X-ray reports from the NIH and the Medical Information Mart for Intensive Care (MIMIC) Database, a publicly available dataset of de-identified electronic health records.
“Preliminary evaluation has shown that Vicuna, a free publicly available LLM, approaches the performance of ChatGPT in tasks such as multi-lingual question answering,” Dr. Summers said.
The study dataset included 3,269 chest X-ray reports obtained from MIMIC and 25,596 reports from the NIH.
Using two prompts for two tasks, the researchers asked the LLM to identify and label the presence or absence of 13 specific findings on the chest X-ray reports. Researchers compared the LLM’s performance with two widely used non-LLM labeling tools.
A statistical analysis of the LLM output showed moderate to substantial agreement with the non-LLM computer programs.
“Our study demonstrated that the LLM’s performance was comparable to the current reference standard,” Dr. Summers said. “With the right prompt and the right task, we were able to achieve agreement with currently used labeling tools.”
Dr. Summers said LLMs that can be run locally will be useful in creating large data sets for AI research without compromising patient privacy.
“LLMs have turned the whole paradigm of natural language processing on its head,” he said. “They have the potential to do things that we've had difficulty doing with traditional pre-large language models.”
Dr. Summers said LLM tools could be used to extract important information from other text-based radiology reports and medical records, and as a tool for identifying disease biomarkers.
“My lab has been focusing on extracting features from diagnostic images,” he said. “With tools like Vicuna, we can extract features from the text and combine them with features from images for input into sophisticated AI models that may be able to answer clinical questions.
“LLMs that are free, privacy-preserving, and available for local use are game changers,” he said. “They're really allowing us to do things that we weren't able to do before.”
“Feasibility of Using the Privacy-preserving Large Language Model Vicuna for Labeling Radiology Reports.” Collaborating with Drs. Summers and Mukherjee were Benjamin Hou, Ph.D., and Ricardo B. Lanfredi, Ph.D.
In 2023, Radiology is celebrating its 100th anniversary with 12 centennial issues, highlighting Radiology’s legacy of publishing exceptional and practical science to improve patient care.
Radiology is edited by Linda Moy, M.D., New York University, New York, N.Y., and owned and published by the Radiological Society of North America, Inc. (https://pubs.rsna.org/journal/radiology)
RSNA is an association of radiologists, radiation oncologists, medical physicists and related scientists promoting excellence in patient care and health care delivery through education, research and technologic innovation. The Society is based in Oak Brook, Illinois. (RSNA.org)
For patient-friendly information on chest X-rays, visit RadiologyInfo.org.
Video (MP4):
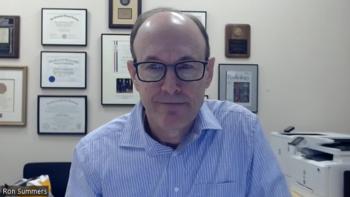
Video 1. Ronald M. Summers, M.D., Ph.D., discusses his research on testing large language models that preserve patient privacy.
Download MP4
(Right-click and Save As)
Images (JPG, TIF):
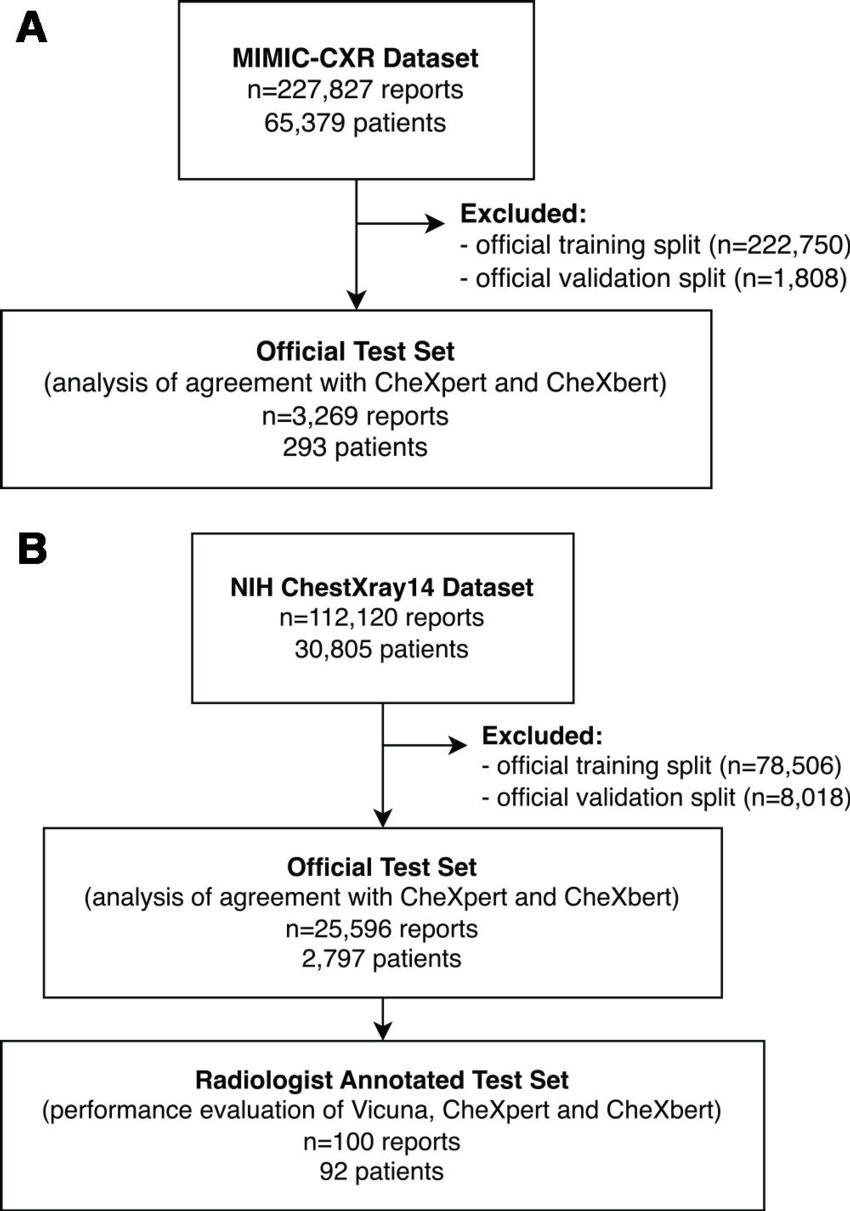
Figure 1. Flowchart shows the data selection process from the (A) MIMIC-CXR and (B) National Institutes of Health (NIH) ChestX-ray14 data sets.
High-res (TIF) version
(Right-click and Save As)
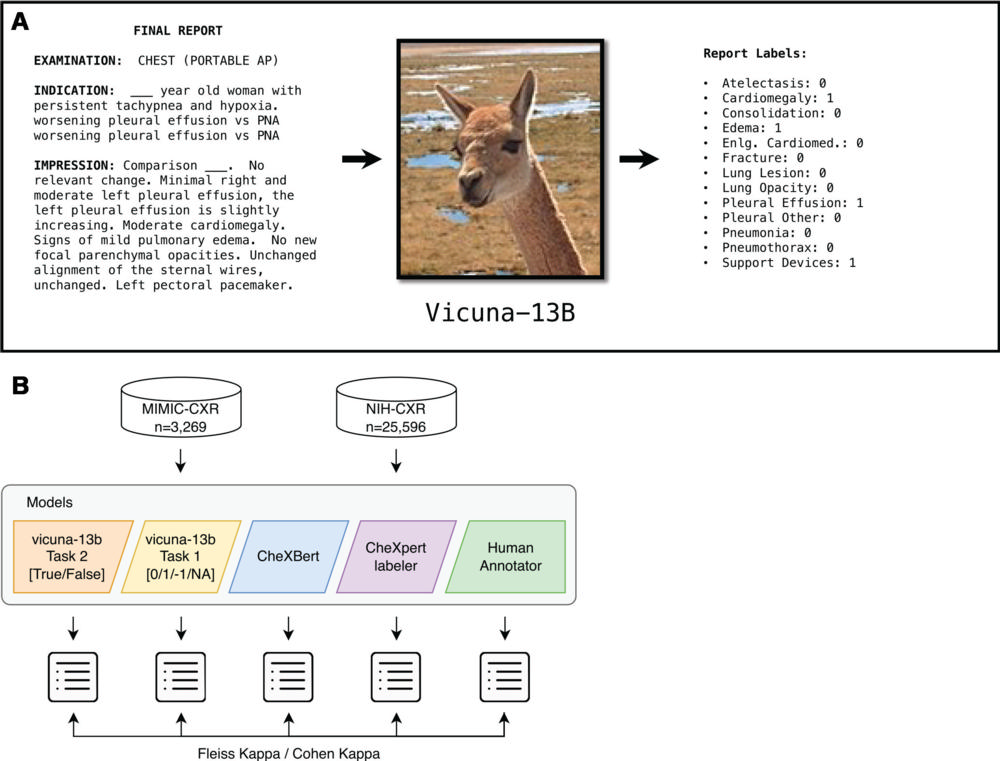
Figure 2. Overview of the study. (A) The open-access large language model Vicuna-13B, which can be run on a local computer without the need for de-identification of patient data, was prompted to examine unstructured, free-text chest radiography (CXR) reports and generate an output file reporting the results of 13 specific findings. AP = anteroposterior, Enlg. Cardiomed. = enlarged cardiomediastinum, PNA = pneumonia. (B) Reports from the MIMIC-CXR data set (n = 3269) and the National Institutes of Health (NIH) data set (n = 25 596) were used in this study. Vicuna was given two independent tasks that generated two different output files, one in which the 13 possible findings were labeled as positive or negative (task 2, orange model) and the other in which the 13 possible findings were labeled as positive, negative, unsure, or not mentioned (task 1, yellow model). The agreement between Vicuna model outputs and the CheXbert labeler, CheXpert labeler, and human annotations were compared using Fleiss or Cohen κ as appropriate.
High-res (TIF) version
(Right-click and Save As)
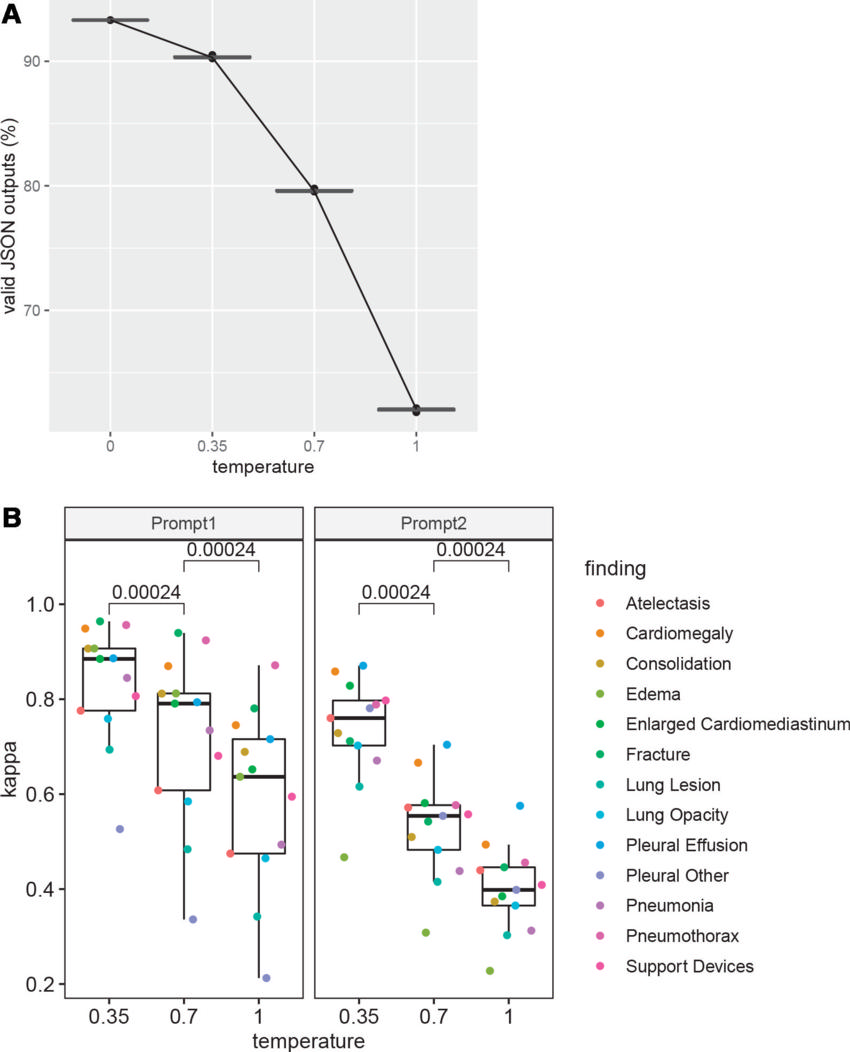
Figure 3. Effect of the temperature hyperparameter. (A) Line graph shows that the proportion of valid JavaScript object notation (JSON) outputs obtained from Vicuna using prompt 1 on the National Institutes of Health data set decreased as the temperature hyperparameter increased. At each of the three temperatures of 0.35, 0.7, and 1, three points are plotted showing the actual proportion of the valid JSON outputs for the three runs. The horizontal lines mark the medians of the three points for each temperature. (B) Box and whisker plots of the degree of agreement measured with use of Fleiss κ among three runs of Vicuna show significant decreases as the temperature increases for both prompt 1 and prompt 2. The numbers near the top of the plot represent P values computed using the Wilcoxon signed-rank test. In this plot, the midline represents the median, and box edges represent the first and third quartiles. The whiskers represent the range of the data, excluding outliers. The outliers are points that are beyond.
High-res (TIF) version
(Right-click and Save As)
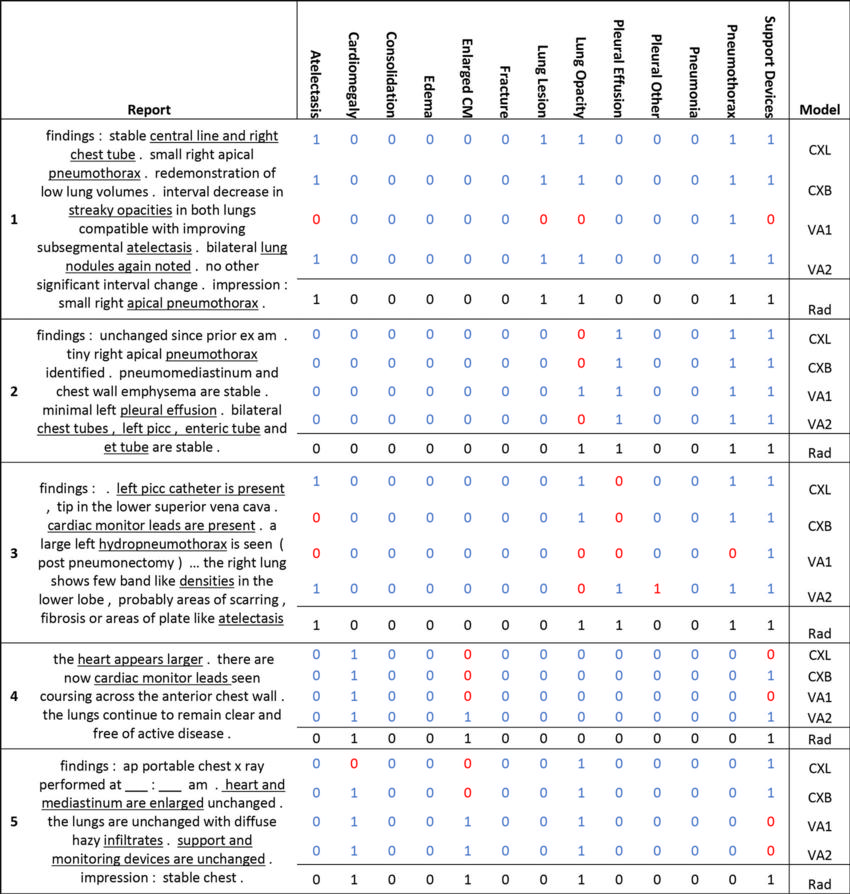
Figure 4. Example chest radiography reports and findings identified by CheXpert, CheXbert, Vicuna, and a radiologist. Both prompt 1 (a single-step prompt) and prompt 2 (a multistep interactive rule-based prompt) were used to run task 2 (positive or negative finding) on the large language model Vicuna. A value of 1 indicates a positive finding, while 0 indicates a negative finding. Values in blue represent outputs that agreed with the findings of the radiologist (Rad) (numbers in black), and red values represent outputs that disagreed with the findings of the radiologist. Key phrases in the reports are underlined. ap = anteroposterior, CM = cardiomediastinum, CXB = CheXbert labeler, CXL = CheXpert labeler, et = endotracheal, exam = examination, picc = peripherally inserted central catheter, VA1 = Vicuna run with prompt 1, VA2 = Vicuna run with prompt 2.
High-res (TIF) version
(Right-click and Save As)