AI Chest X-ray Model Analysis Reveals Race and Sex Bias
Released: September 27, 2023
At A Glance
- AI chest X-ray foundation model analysis revealed differences in performance across patient subgroups.
- Researchers compared the performance of a chest X-ray foundation model and a reference model built by the team in evaluating 127,118 chest X-rays.
- Significant differences were found between male and female and Asian and Black patients in the features related to disease detection.
- RSNA Media Relations
1-630-590-7762
media@rsna.org - Linda Brooks
1-630-590-7738
lbrooks@rsna.org - Imani Harris
1-630-481-1009
iharris@rsna.org
OAK BROOK, Ill. — An AI chest X-ray foundation model for disease detection demonstrated racial and sex-related bias leading to uneven performance across patient subgroups and may be unsafe for clinical applications, according to a study published today in Radiology: Artificial Intelligence, a journal of the Radiological Society of North America (RSNA). The study aims to highlight the potential risks for using foundation models in the development of medical imaging artificial intelligence.
"There's been a lot of work developing AI models to help doctors detect disease in medical scans," said lead researcher Ben Glocker, Ph.D., professor of machine learning for imaging at Imperial College London in the U.K. "However, it can be quite difficult to get enough training data for a specific disease that is representative of all patient groups."
Due to the difficulty of collecting large volumes of high-quality training data, the AI field has moved toward using deep-learning foundation models that have been trained for other purposes. Foundation models are AI neural networks that have been trained on large, often unlabeled datasets which handle jobs from translating text to analyzing medical images.
"Despite their increasing popularity, we know little about potential biases in foundation models that could affect downstream uses," Dr. Glocker said.
Dr. Glocker's research team compared the performance of a recently published chest X-ray foundation model and a reference model built by the team in evaluating 127,118 chest X-rays with associated diagnostic labels. The pre-trained foundation model was built with more than 800,000 chest X-rays from India and the U.S.
The researchers completed a comprehensive performance analysis to determine how well the models performed for individual subgroups. The 42,884 patients (mean age, 63; 23,623 male) in the study group included Asian, Black and white patients.
Bias analysis showed significant differences between features related to disease detection across biological sex and race.
"Our bias analysis showed that the foundation model consistently underperformed compared to the reference model," Dr. Glocker said. "We observed a decline in disease classification performance and specific disparities in protected subgroups."
Significant differences were found between male and female and Asian and Black patients in the features related to disease detection. Compared with the average model performance across all subgroups, classification performance on the 'no finding' label dropped between 6.8% and 7.8% for female patients, and performance in detecting 'pleural effusion'—a buildup of fluid around the lungs—dropped between 10.7% and 11.6% for Black patients.
"Dataset size alone does not guarantee a better or fairer model," Dr. Glocker said. "We need to be very careful about data collection to ensure diversity and representativeness."
He noted that it's important that foundation models are published and shared.
"To minimize the risk of bias associated with the use of foundation models for clinical decision-making, these models need to be fully accessible and transparent," he said.
Dr. Glocker is an advocate for comprehensive bias analysis as an integral part of the development and auditing of foundation models.
"AI is often seen as a black box, but that's not entirely true," he said. "We can open the box and inspect the features. Model inspection is one way of continuously monitoring and flagging issues that need a second look."
The work doesn't start with the AI model, it starts with the data used to build it, Dr. Glocker noted.
"As we collect the next dataset, we need to, from day one, make sure AI is being used in a way that will benefit everyone," he said.
"Risk of Bias in Chest Radiography Deep Learning Foundation Models." Collaborating with Dr. Glocker were Charles Jones, M.Eng., Mélanie Roschewitz, M.Sc., and Stefan Winzeck, Ph.D.
Radiology: Artificial Intelligence is edited by Charles E. Kahn Jr., M.D., M.S., Perelman School of Medicine at the University of Pennsylvania, and owned and published by the Radiological Society of North America, Inc. (https://pubs.rsna.org/journal/ai)
RSNA is an association of radiologists, radiation oncologists, medical physicists and related scientists promoting excellence in patient care and health care delivery through education, research and technologic innovation. The Society is based in Oak Brook, Illinois. (RSNA.org)
For patient-friendly information on chest X-rays, visit RadiologyInfo.org.
Video (MP4):
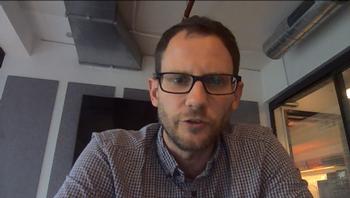
Video 1. Ben Glocker, Ph.D., discusses his research: AI Chest X-ray Model Analysis Reveals Race and Sex Bias
Download MP4
(Right-click and Save As)
Images (JPG, TIF):
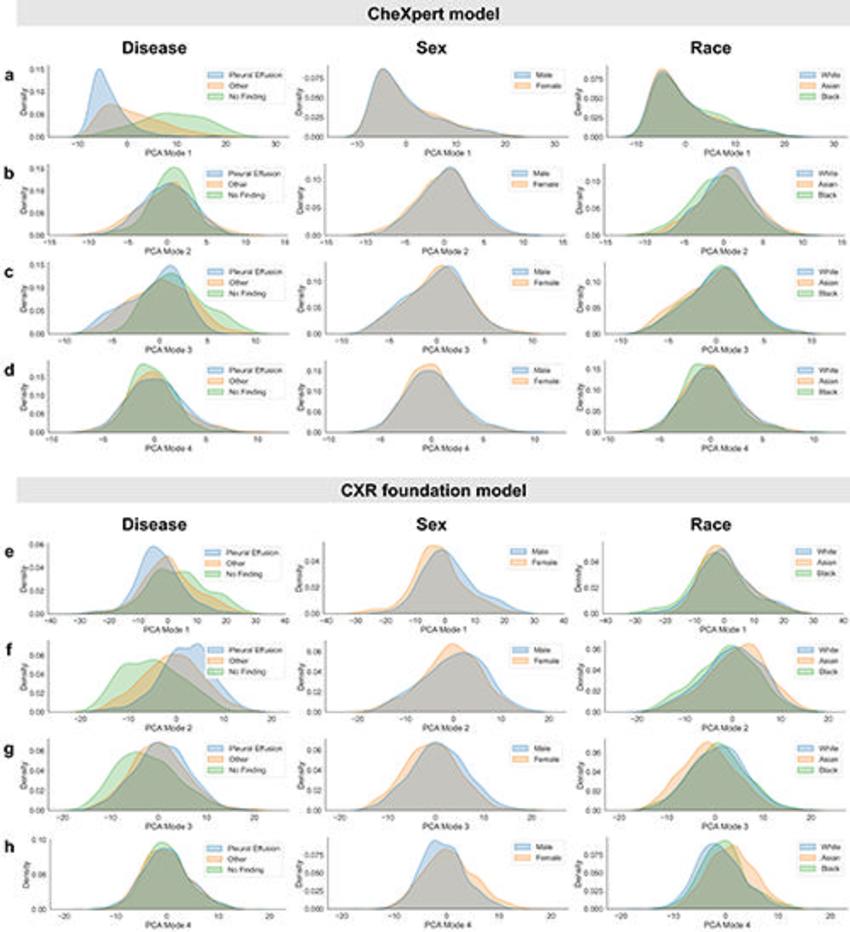
Figure 1. Inspection of subgroup distribution shifts in the PCA feature space projections. Marginal distributions are plotted across subgroups for the first four modes of PCA applied to the extracted feature vectors of the CheXpert test data for (A–D) the CheXpert model and (E–H) the CXR foundation model. The plots are generated using a random set of 3,000 patients (1,000 samples from each racial group). Marginal distributions are normalized independently to remove differences in subgroup base rates and shown for different characteristics (from left to right): presence of disease, biologic sex, and racial identity. Larger distribution shifts across sex and race are observed for the CXR foundation model. CXR = chest radiography, PCA = principal component analysis.
High-res (TIF) version
(Right-click and Save As)
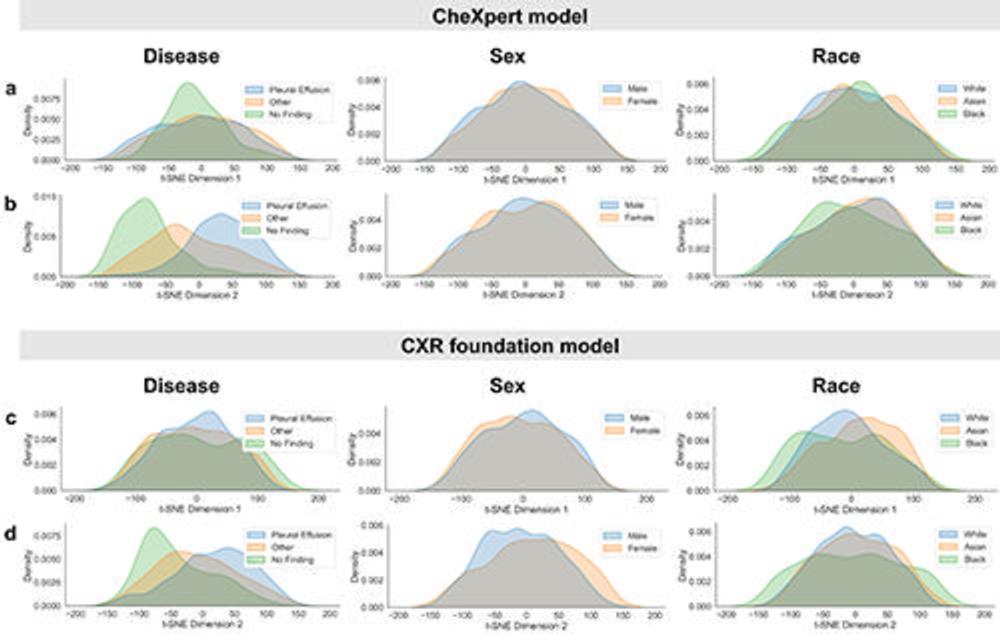
Figure 2. Inspection of subgroup distribution shifts in the t-SNE feature space projections. Marginal distributions are plotted across subgroups for the two dimensions of t-SNE applied to the extracted feature vectors of the CheXpert test data for (A, B) the CheXpert model and (C, D) the CXR foundation model. The plots are generated using a random set of 3,000 patients (1,000 samples from each racial group). Marginal distributions are normalized independently to remove differences in subgroup base rates and shown for different characteristics (from left to right): presence of disease, biologic sex, and racial identity. Larger distribution shifts across sex and race are observed for the CXR foundation model. CXR = chest radiography, t-SNE = t-distributed stochastic neighbor embedding.
High-res (TIF) version
(Right-click and Save As)
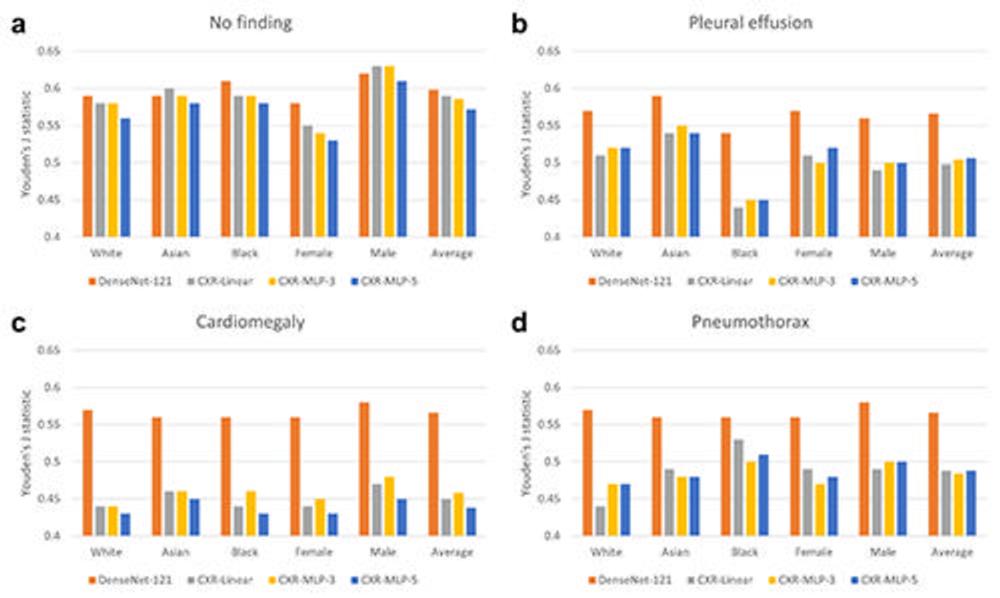
Figure 3. Comparison of disease detection performance across patient subgroups. Average classification performance across patient subgroups is shown in terms of Youden’s J statistic for the DenseNet-121 CheXpert model and three variants of the CXR foundation model. Classification performance is shown on four different labels of (A) ‘no finding’, (B) ‘pleural effusion’, (C) ‘cardiomegaly’, and (D) ‘pneumothorax’. The CXR foundation models consistently underperformed compared with the CheXpert model, with specific underperformance on the subgroup of female patients for ‘no finding’ and the subgroup of Black patients on ‘pleural effusion’. There was also a drastic decrease in overall performance across all subgroups for the CXR foundation models for ‘cardiomegaly’. CXR = chest radiography, MLP = multilayer perceptrons.
High-res (TIF) version
(Right-click and Save As)
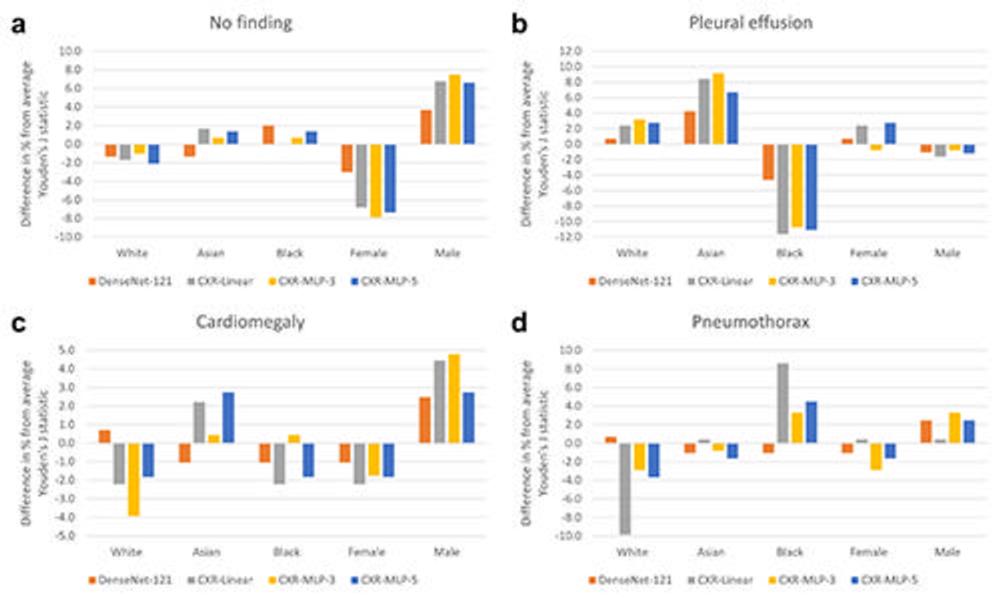
Figure 4. Relative change in disease detection performance across patient subgroups. The relative change in performance for each subgroup was measured by comparing the subgroup performance with each model’s average performance over all subgroups. Performance is measured in terms of Youden’s J statistic on the labels of (A) ‘no finding’, (B) ‘pleural effusion’, (C) ‘cardiomegaly’, and (D) ‘pneumothorax’. There were substantially larger disparities in relative performance across biologic sex and race for the three CXR foundation models, CXR-Linear, CXR-MLP-3, and CXR-MLP-5 when compared with the DenseNet-121 CheXpert model. CXR = chest radiography, MLP = multilayer perceptrons.
High-res (TIF) version
(Right-click and Save As)