Researchers Use AI to Triage Patients with Chest Pain
Released: January 17, 2023
At A Glance
- Deep learning analysis of initial chest X-rays may facilitate more efficient triage of patients arriving at the ER with acute chest pain.
- The deep-learning model significantly improved prediction of acute coronary syndrome, pulmonary embolism and aortic dissection.
- Acute chest pain syndrome accounts for over 7 million emergency department visits annually in the United States, making it one of the most common complaints.
- RSNA Media Relations
1-630-590-7762
media@rsna.org - Linda Brooks
1-630-590-7738
lbrooks@rsna.org - Imani Harris
1-630-481-1009
iharris@rsna.org
OAK BROOK, Ill. (January 17, 2023) — Artificial intelligence (AI) may help improve care for patients who show up at the hospital with acute chest pain, according to a study published in Radiology, a journal of the Radiological Society of North America (RSNA).
"To the best of our knowledge, our deep learning AI model is the first to utilize chest X-rays to identify individuals among acute chest pain patients who need immediate medical attention," said the study's lead author, Márton Kolossváry, M.D., Ph.D., radiology research fellow at Massachusetts General Hospital (MGH) in Boston.
Acute chest pain syndrome may consist of tightness, burning or other discomfort in the chest or a severe pain that spreads to your back, neck, shoulders, arms, or jaw. It may be accompanied by shortness of breath.
Acute chest pain syndrome accounts for over 7 million emergency department visits annually in the United States, making it one of the most common complaints.
Fewer than 8% of these patients are diagnosed with the three major cardiovascular causes of acute chest pain syndrome, which are acute coronary syndrome, pulmonary embolism or aortic dissection. However, the life-threatening nature of these conditions and low specificity of clinical tests, such as electrocardiograms and blood tests, lead to substantial use of cardiovascular and pulmonary diagnostic imaging, often yielding negative results. As emergency departments struggle with high patient numbers and shortage of hospital beds, effectively triaging patients at very low risk of these serious conditions is important.
Deep learning is an advanced type of artificial intelligence (AI) that can be trained to search X-ray images to find patterns associated with disease.
For the study, Dr. Kolossváry and colleagues developed an open-source deep learning model to identify patients with acute chest pain syndrome who were at risk for 30-day acute coronary syndrome, pulmonary embolism, aortic dissection or all-cause mortality, based on a chest X-ray.
The study used electronic health records of patients presenting with acute chest pain syndrome who had a chest X-ray and additional cardiovascular or pulmonary imaging and/or stress tests at MGH or Brigham and Women's Hospital in Boston between January 2005 and December 2015. For the study, 5,750 patients (mean age 59, including 3,329 men) were evaluated.
The deep-learning model was trained on 23,005 patients from MGH to predict a 30-day composite endpoint of acute coronary syndrome, pulmonary embolism or aortic dissection and all-cause mortality based on chest X-ray images.
The deep-learning tool significantly improved prediction of these adverse outcomes beyond age, sex and conventional clinical markers, such as d-dimer blood tests. The model maintained its diagnostic accuracy across age, sex, ethnicity and race. Using a 99% sensitivity threshold, the model was able to defer additional testing in 14% of patients as compared to 2% when using a model only incorporating age, sex, and biomarker data.
"Analyzing the initial chest X-ray of these patients using our automated deep learning model, we were able to provide more accurate predictions regarding patient outcomes as compared to a model that uses age, sex, troponin or d-dimer information," Dr. Kolossváry said. "Our results show that chest X-rays could be used to help triage chest pain patients in the emergency department."
According to Dr. Kolossváry, in the future such an automated model could analyze chest X-rays in the background and help select those who would benefit most from immediate medical attention and may help identify patients who may be discharged safely from the emergency department.
"Deep Learning Analysis of Chest Radiographs to Triage Patients with Acute Chest Pain Syndrome." Collaborating with Dr. Kolossváry were Vineet K. Raghu, Ph.D., John T. Nagurney, M.D., Udo Hoffmann, M.D., M.P.H., and Michael T. Lu, M.D., M.P.H.
In 2023, Radiology is celebrating its 100th anniversary with 12 centennial issues, highlighting Radiology's legacy of publishing exceptional and practical science to improve patient care.
Radiology is edited by Linda Moy, M.D., New York University, New York, N.Y., and owned and published by the Radiological Society of North America, Inc. (https://pubs.rsna.org/journal/radiology)
RSNA is an association of radiologists, radiation oncologists, medical physicists and related scientists promoting excellence in patient care and health care delivery through education, research, and technologic innovation. The Society is based in Oak Brook, Illinois. (RSNA.org)
For patient-friendly information on chest X-rays, visit RadiologyInfo.org.
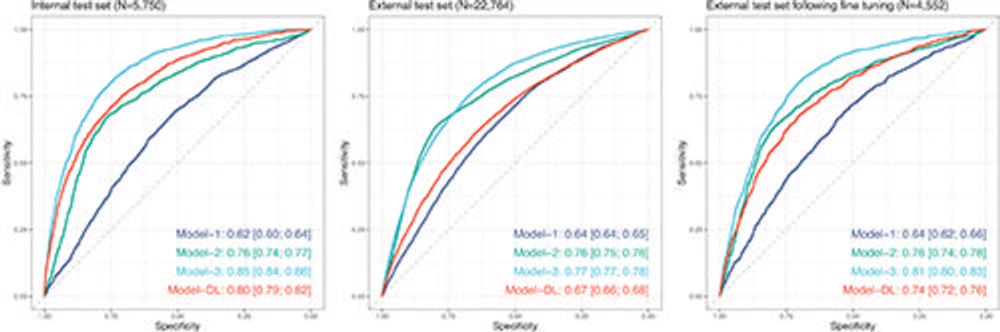
Figure 1. Diagnostic performance of the models to predict the composite end point within 30 days of emergency department (ED) registration. Model 1, age + sex; model 2, model 1 + serum biomarker positivity; model 3, model 2 + deep learning (DL) predictions from chest radiographs; model DL, DL predictions from chest radiographs. All statistical comparisons between area under the receiver operating characteristic curve values of models 1–3 were significant (P < .001). Data in brackets are 95% CIs. Composite end point was defined as follows: acute coronary syndrome or pulmonary embolism or aortic dissection or all-cause mortality within 30 days of ED registration.
High-res (TIF) version
(Right-click and Save As)
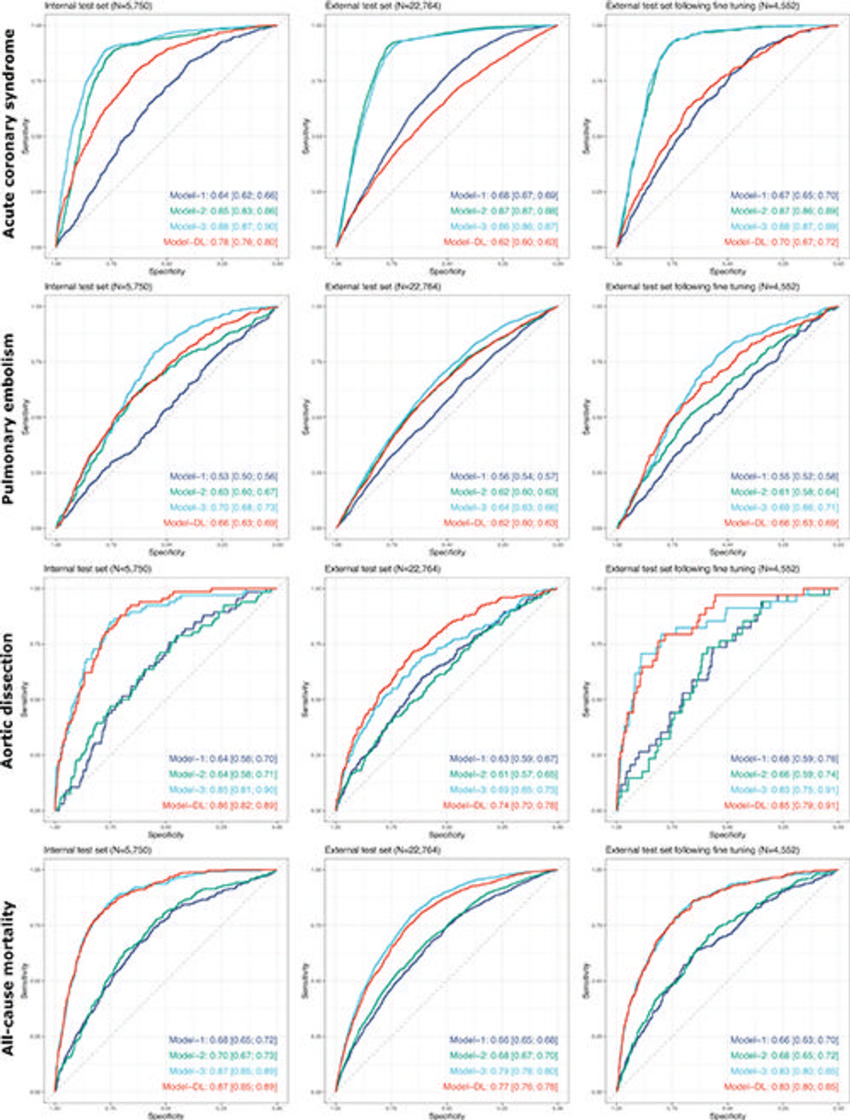
Figure 2. Diagnostic performance of the models in predicting specific 30-day clinical end points. Data are area under the receiver operating characteristic curve, with the 95% CI in brackets. Model 1, age + sex; model 2, model 1 + serum biomarker positivity; model 3, model 2 + deep learning (DL) predictions from chest radiographs; model DL, DL predictions from chest radiographs. Diagnostic performance of the models in predicting specific 30-day clinical end points. Data are area under the receiver operating characteristic curve, with the 95% CI in brackets. Model 1, age + sex; model 2, model 1 + serum biomarker positivity; model 3, model 2 + DL predictions from chest radiographs; model DL, DL predictions from chest radiographs.
High-res (TIF) version
(Right-click and Save As)
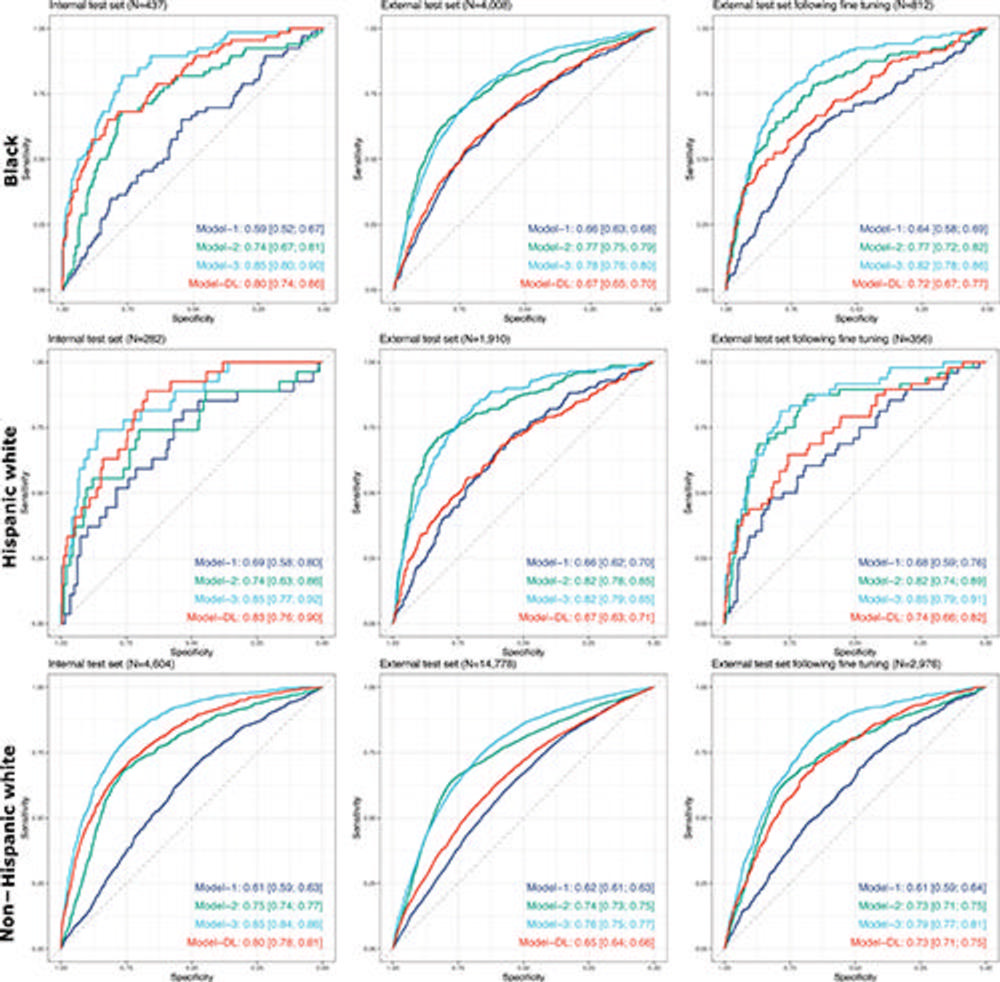
Figure 3. Diagnostic performance of the models in predicting the 30-day composite end point stratified by race and ethnicity. Data are area under the receiver operating characteristic (AUC) curve, with the 95% CI in brackets. Model 1, age + sex; model 2, model 1 + serum biomarker positivity; model 3, model 2 + deep learning (DL) predictions from chest radiographs; model DL, DL predictions from chest radiographs. All statistical comparisons between the AUC values of models 1–3 were significant (P < .001). Composite end point was defined aortic dissection or all-cause mortality within 30 days of emergency department registration.
High-res (TIF) version
(Right-click and Save As)
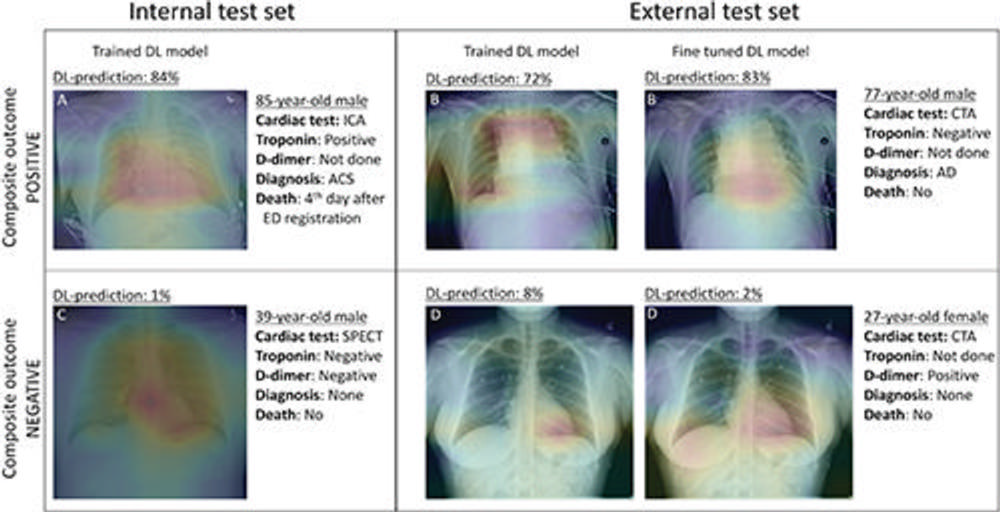
Figure 4. Gradient-weighted class activation maps of representative chest radiographs in (A) an 85-year-old man with acute coronary syndrome (ACS), (B) a 77-year-old man with aortic dissection (AD), (C) a 39-year-old healthy man, and (D) a 27-year-old healthy woman. The maps show which parts of the images influenced deep learning (DL) model predictions for the composite outcome. The color gradient shows the level of activation from that given area, where red indicates the highest activation, blue indicates the lowest activation, and no color indicates no activation. Areas of the heart and lungs contributed most model predictions. Fine-tuning improved the diagnostic accuracy of our DL model and resulted in more relevant areas contributing to predictions. ACS = acute coronary syndrome, CTA = coronary CT angiography, ICA = invasive coronary angiography, SPECT = single-photon emission CT.
High-res (TIF) version
(Right-click and Save As)