Eye Exam Could Lead to Early Parkinson’s Disease Diagnosis
Released: November 23, 2020
At A Glance
- Artificial intelligence and a simple eye exam could provide early diagnosis of Parkinson’s disease.
- Using pictures of the back of the eye, researchers trained a type of AI called support vector machine learning to detect signs suggestive of disease.
- The results indicated that the machine learning networks can classify Parkinson’s disease based on retina vasculature, with the key features being smaller blood vessels.
- RSNA Media Relations
1-630-590-7762
media@rsna.org - Linda Brooks
1-630-590-7738
lbrooks@rsna.org
OAK BROOK, Ill. — A simple eye exam combined with powerful artificial intelligence (AI) machine learning technology could provide early detection of Parkinson’s disease, according to research being presented at the annual meeting of the Radiological Society of North America (RSNA).
Parkinson’s disease is a progressive disorder of the central nervous system that affects millions of people worldwide. Diagnosis is typically based on symptoms like tremors, muscle stiffness and impaired balance—an approach that has significant limitations.
“The issue with that method is that patients usually develop symptoms only after prolonged progression with significant injury to dopamine brain neurons,” said study lead author Maximillian Diaz, a biomedical engineering Ph.D. student at the University of Florida in Gainesville, Florida. “This means that we are diagnosing patients late in the disease process.”
Disease progression is characterized by nerve cell decay that thins the walls of the retina, the layer of tissue that lines the back of the eyeball. The disease also affects the microscopic blood vessels, or microvasculature, of the retina. These characteristics present an opportunity to leverage the power of AI to examine images of the eyes for signs of Parkinson’s disease.
For the new study, Diaz collaborated with graduate student Jianqiao Tian and University of Florida neurologist Adolfo Ramirez-Zamora, M.D., under the direction of Ruogu Fang, Ph.D., director of the J. Crayton Pruitt Department of Biomedical Engineering’s Smart Medical Informatics Learning and Evaluation Lab (SMILE).
The researchers deployed a type of AI called support vector machine (SVM) learning that has been around since 1989. Using pictures of the back of the eye from both patients with Parkinson’s disease and control participants, they trained the SVM to detect signs on the images suggestive of disease.
The results indicated that the machine learning networks can classify Parkinson’s disease based on retina vasculature, with the key features being smaller blood vessels. The proposed methods further support the idea that changes in brain physiology can be observed in the eye.
“The single most important finding of this study was that a brain disease was diagnosed with a basic picture of the eye,” Diaz said. “This is very different from traditional approaches where to find a problem with the brain you look at different brain images.”
Diaz noted that those traditional imaging approaches with MRI, CT and nuclear medicine techniques can be very costly. In contrast, the new approach uses basic photography with equipment commonly available in eye clinics to get an image. The images can even be captured by a smartphone with a special lens.
“It’s just a simple picture of the eye, you can have it done in less than a minute, and the cost of the equipment is much less than a CT or MRI machine,” Diaz said. “If we can make this a yearly screening, then the hope is that we can catch more cases sooner, which can help us better understand the disease and find a cure or a way to slow the progression.”
The approach may also have applications in identifying other diseases that affect the structure of the brain, such as Alzheimer’s disease and multiple sclerosis, Diaz said.
For more information and images, visit RSNA.org/press20. Press account required to view embargoed materials.
RSNA is an association of radiologists, radiation oncologists, medical physicists and related scientists promoting excellence in patient care and health care delivery through education, research and technologic innovation. The Society is based in Oak Brook, Illinois. (RSNA.org)
Editor’s note: The data in these releases may differ from those in the published abstract and those actually presented at the meeting, as researchers continue to update their data right up until the meeting. To ensure you are using the most up-to-date information, please call the RSNA media relations team at Newsroom at 1-630-590-7762.
For patient-friendly information on Parkinson’s disease, visit RadiologyInfo.org.
Video (MP4):
Images (JPG, TIF):
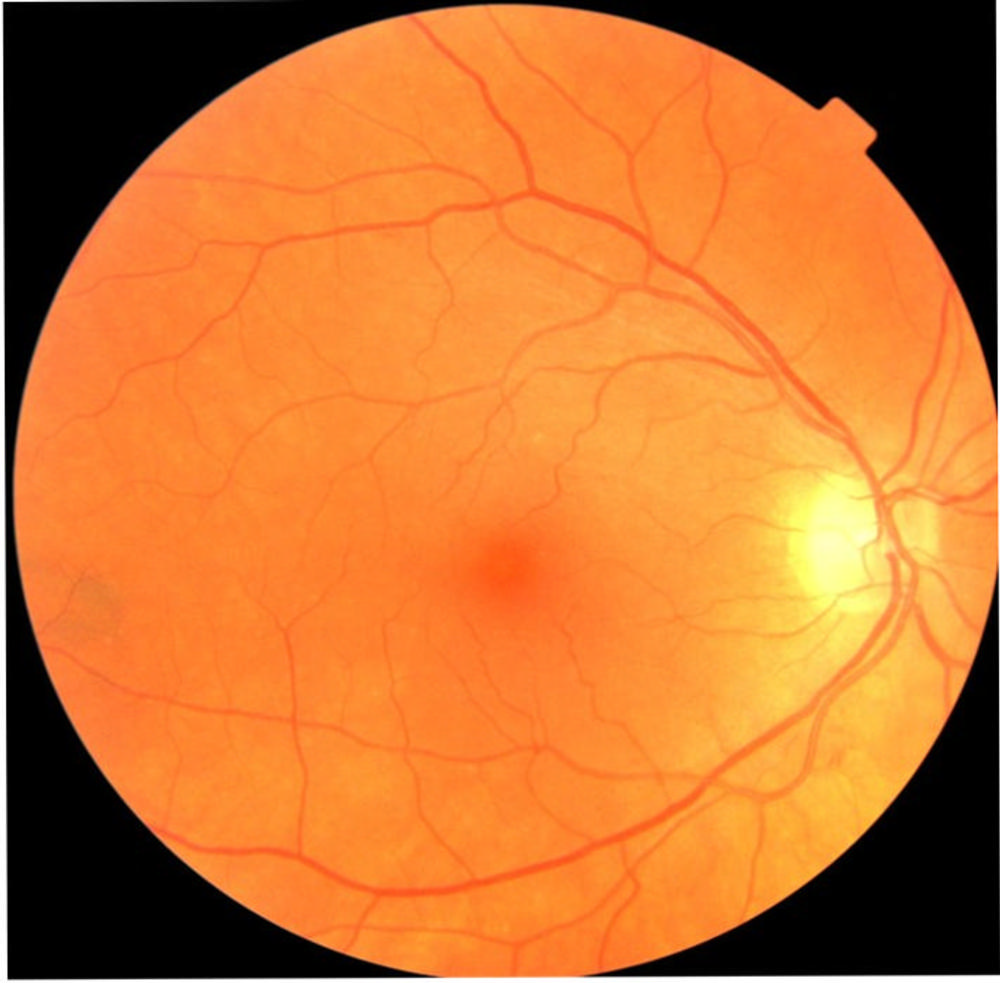
Figure 1. An example of a fundus eye images taken from the UK Biobank.
High-res (TIF) version
(Right-click and Save As)
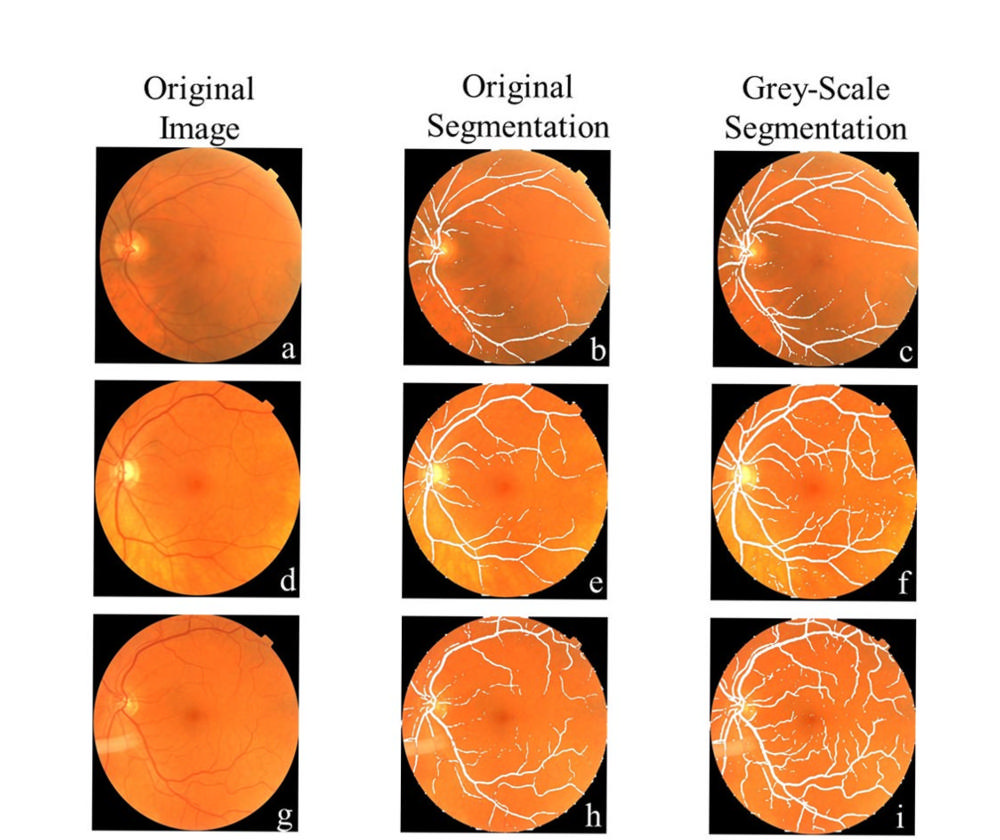
Figure 2. Comparison of vessel segmentation from input image: (a-c) The grey-scale method fully selected the primary vessel running horizontally along the image, when the original selection only obtained small sections. (d-f) Improved vessel selection could segment select smaller vessels found throughout the image, although it was sometimes limited to broken-up sections. (g-i) A representation of the best-case vessel selection where all the vessels found in the image are selected.
High-res (TIF) version
(Right-click and Save As)
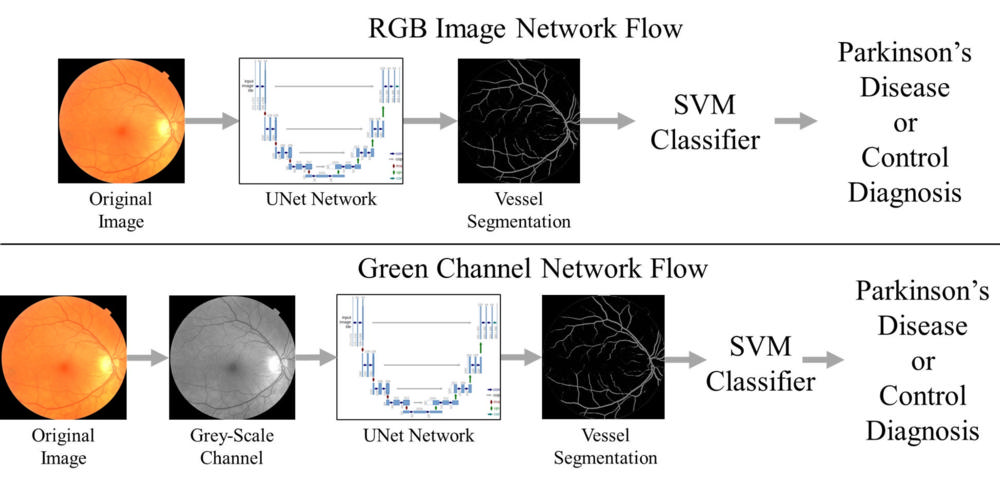
Figure 3. Machine learning processes: (a) The blood vessels are selected from the original fundus image by a machine learning network called U-Net which was trained to select blood vessels in the eye. The vessels are then fed into the support vector machine classifier to diagnose if a person has Parkinson’s disease. (b) A grey-scale image is created from the fundus image and them the vessels are selected by the same U-Net network to improve the selection of blood vessels.
High-res (TIF) version
(Right-click and Save As)
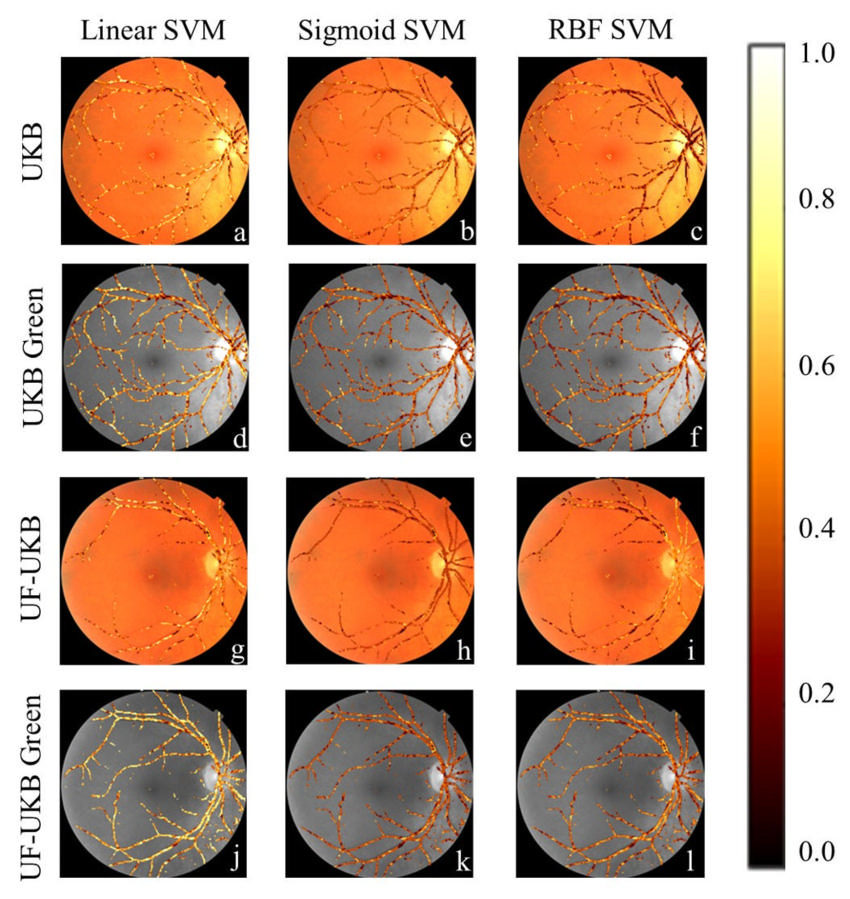
Figure 4. Regions of importance for support vector machine (SVM) classifiers: (a,d,g,j) The linear SVM classifier placed higher importance on most vessel pixels regardless of the data set used. This meant that it focused on both the large and small vessels. (b,e,h,k) Sigmoid SVM classifiers were more selective, in which pixels it placed importance on, with a greater importance on smaller blood vessels, and staggered importance on the large vessels. (c,f,i,l) SVM classifiers developed with an radial base function kernel showed greater variety on which vessels it placed the highest importance between the different datasets. For the UK Biobank (UKB) dataset it focused on smaller blood vessels, but for the University of Florida-UKB (UF-UKB) dataset it placed the focus on the major vessels. In contrast both the UKB Green and UF-UKB Green datasets had a more distributed importance with a bias to either the minor or major vessels.
High-res (TIF) version
(Right-click and Save As)
Powerpoint: