AI Tool Improves Breast Cancer Detection on Mammography
Released: November 04, 2020
At A Glance
- AI can improve the performance of radiologists in reading breast cancer screening mammograms.
- Radiologists assessed a dataset of 240 digital mammography images that included different types of abnormalities.
- AI helped increase the average sensitivity for cancer and reduced the rate of false negatives.
- RSNA Media Relations
1-630-590-7762
media@rsna.org - Linda Brooks
1-630-590-7738
lbrooks@rsna.org
OAK BROOK, Ill. — Artificial intelligence (AI) can enhance the performance of radiologists in reading breast cancer screening mammograms, according to a study published in Radiology: Artificial Intelligence.
Breast cancer screening with mammography has been shown to improve prognosis and reduce mortality by detecting disease at an earlier, more treatable stage. However, many cancers are missed on screening mammography, and suspicious findings often turn out to be benign. An earlier study from Radiology found that, on average, only 10% of women recalled from screening for additional diagnostic workup based on suspicious findings are ultimately found to have cancer.
AI-based algorithms represent a promising avenue for improving the accuracy of digital mammography. Developers “train” the AI on existing images, teaching it to recognize abnormalities associated with cancer and distinguish them from benign findings. The programs can then be tested on different sets of images. AI offers not only the possibility of better cancer detection but also improved efficiency for radiologists.
For the study, researchers used MammoScreen, an AI tool that can be applied with mammography to aid in cancer detection. The AI system is designed to identify regions suspicious for breast cancer on 2D digital mammograms and assess their likelihood of malignancy. The system takes as input the complete set of four views composing a mammogram and outputs a set of image positions with a related suspicion score.
Fourteen radiologists assessed a dataset of 240 2D digital mammography images acquired between 2013 and 2016 that included different types of abnormalities. Half of the dataset was read without AI and the other half with the help of AI during a first session and without during a second session.
Average sensitivity for cancer increased slightly when using AI support. AI also helped reduce the rate of false negatives, or findings that look normal even though cancer is present.
“The results show that MammoScreen may help to improve radiologists’ performance in breast cancer detection,” said Serena Pacilè, Ph.D., clinical research manager at Therapixel, where the software was developed.
The improved diagnostic performance of radiologists in the detection of breast cancer was achieved without prolonging their workflow. In cases with a low likelihood of malignancy, reading time decreased in the second reading session. This reduced reading time could increase overall radiologists’ efficiency, allowing them to focus their attention on the more suspicious examinations, the researchers said.
In March, the U.S. Food and Drug Administration cleared MammoScreen for use in the clinic, where it could help reduce the workload of radiologists, according to Dr. Pacilè.
The researchers plan to explore the behavior of the AI tool on a large screening-based population and its ability to detect breast cancer earlier.
“Improving Breast Cancer Detection Accuracy of Mammography with the Concurrent Use of an Artificial Intelligence Tool.” Collaborating with Dr. Pacilè were January Lopez, M.D., Pauline Chone, M.Phil., Thomas Bertinotti, M.Sc., Jean Marie Grouin, Ph.D., and Pierre Fillard, Ph.D.
Radiology: Artificial Intelligence is edited by Charles E. Kahn Jr., M.D., M.S., Perelman School of Medicine at the University of Pennsylvania, and owned and published by the Radiological Society of North America, Inc. (https://pubs.rsna.org/journal/ai)
RSNA is an association of radiologists, radiation oncologists, medical physicists and related scientists promoting excellence in patient care and health care delivery through education, research and technologic innovation. The Society is based in Oak Brook, Ill. (RSNA.org)
For patient-friendly information on mammography, visit RadiologyInfo.org.
Images (JPG, TIF):
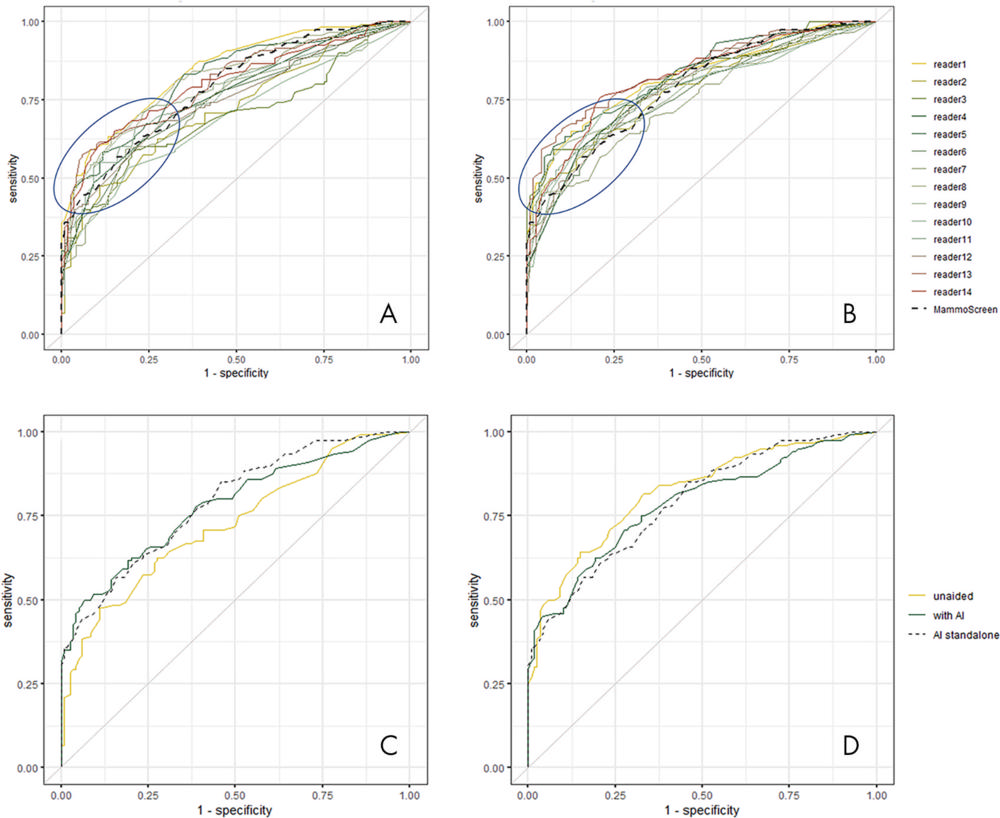
Figure 2. A, Receiver operating characteristic (ROC) curves of all readers in unaided reading condition and, B, reading with the help of artificial intelligence (AI). The blue circle underlines the area where ROC curves are most improved. Examples of ROC curve of, C, reader 2 and, D, reader 11 with and without using the help of AI.
High-res (TIF) version
(Right-click and Save As)
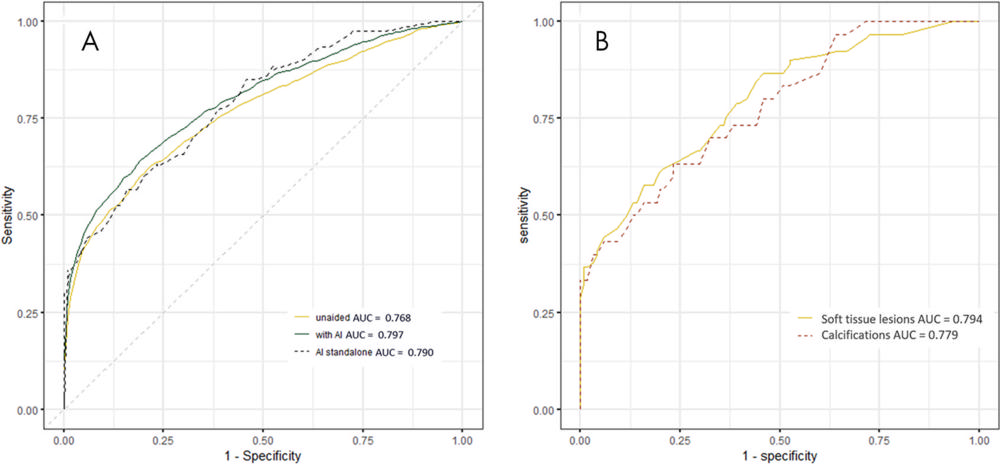
Figure 3. A, Average receiver operating characteristic (ROC) curves of all readers when unassisted (yellow) and assisted with artificial intelligence (AI) (dark green) and ROC curve of the AI system as stand-alone (dashed black). ROC curves are averaged using linear interpolation between sampled points of each curve (the area under the ROC curve [AUC] of the average ROC curve is similar to the average area under the curve of all readers [difference of 1 × 10−3]). B, ROC curve of the AI as a stand-alone system for soft-tissue lesions (yellow) and calcifications (red).
High-res (TIF) version
(Right-click and Save As)
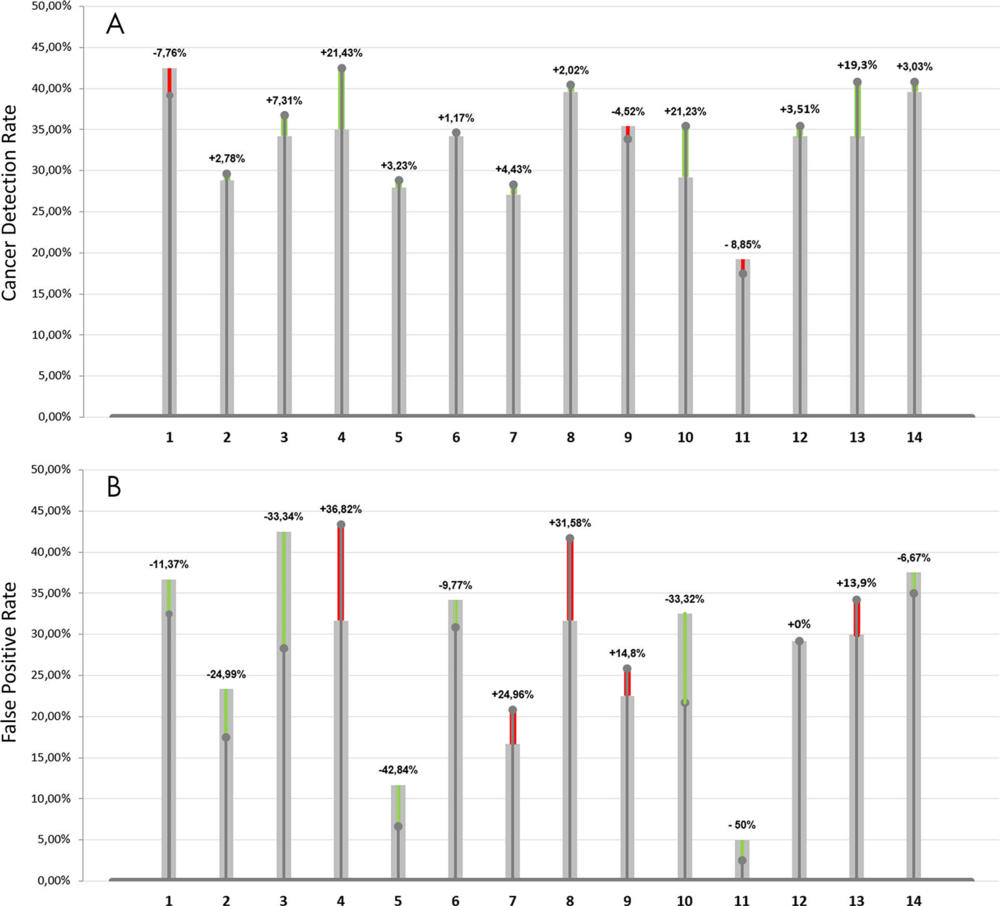
Figure 4. A, Cancer detection rate and percentage improvement brought by the use of the artificial intelligence (AI) system and, B, false-positive rate and percentage decrease as a result of the use of AI. Green bars indicate the percentage improvement brought by the help of AI, thus an increase in cancer detection rate and a decrease in the false-positive rate. Similarly, red bars indicate a deterioration of performances, thus a, A, decrease in cancer detection rate, B, and an increase in false-positive rate.
High-res (TIF) version
(Right-click and Save As)
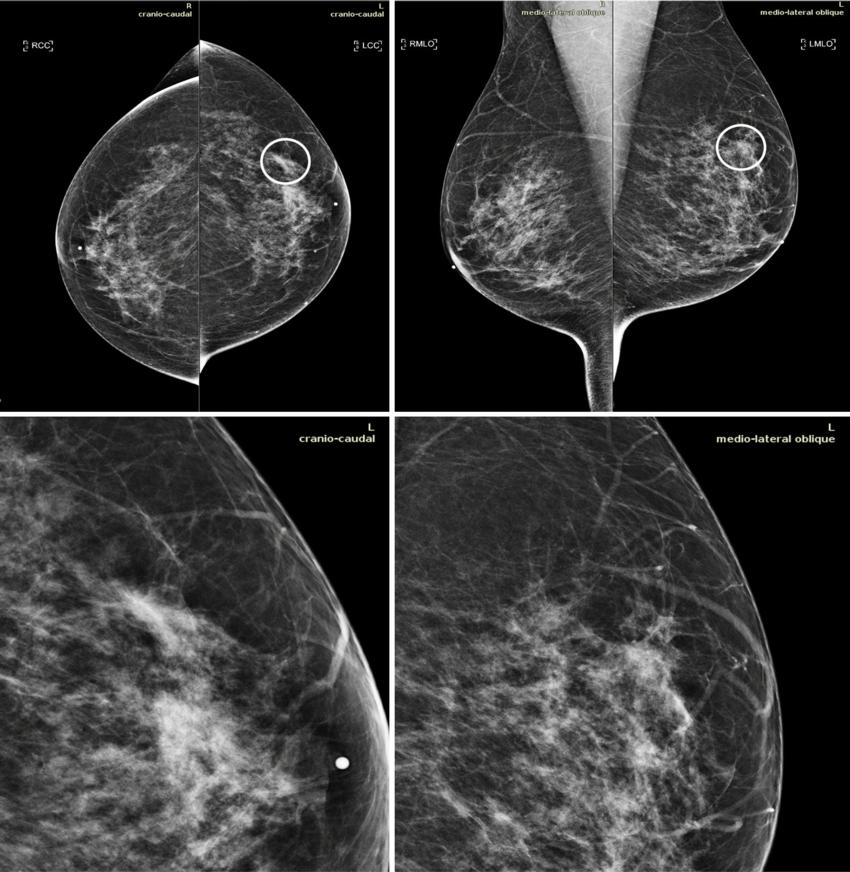
Figure 5. Mammograms in a 51-year-old woman with invasive ductal carcinoma. The upper panels show the craniocaudal and the mediolateral oblique views. The lower panels show a close-up of the left breast area containing the lesion. The case is one of the false-negative cases included in the dataset. Accordingly, the initial screening assessment was a BI-RADS 2, meaning visible findings were judged as benign. After 1 year, the patient presented for another screening examination. This time, a focal asymmetry with associated distortion within the left breast was noticed; the patient was recalled and diagnosed with a 1.5-cm mass in the upper outer quadrant of the left breast on the craniocaudal view (circle).
High-res (TIF) version
(Right-click and Save As)
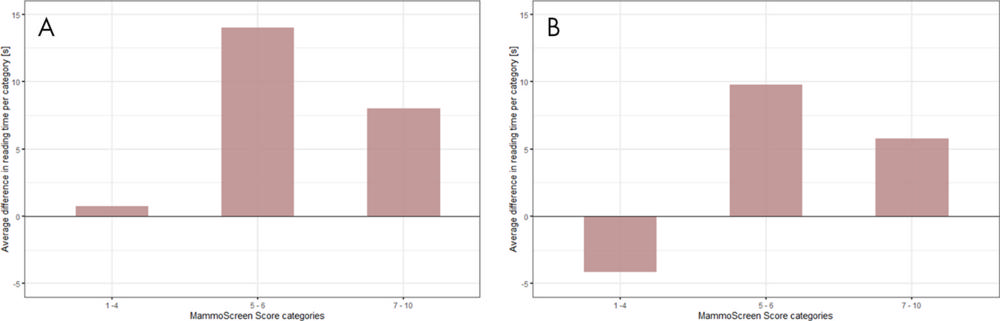
Figure 6. Difference in reading time per MammoScreen for the, A, first reading session and the, B, second reading session. The upper part of the plot indicates an increment in reading time when using MammoScreen. The lower part of the plot indicates a decreased reading time when using MammoScreen. The maximum increase in reading time for the first reading session does not exceed 15 seconds. A session effect is noticeable with a gain in reading time for low MammoScreen score (1 to 4), while the increase does not exceed 10 seconds for the higher scores.
High-res (TIF) version
(Right-click and Save As)