Artificial Intelligence May Help Reduce Gadolinium Dose in MRI
Released: November 26, 2018
At A Glance
- A deep learning algorithm may allow for gadolinium dose reduction in MRI exams.
- Image quality was not significantly different between full-dose, contrast-enhanced images and low-dose images enhanced by the algorithm.
- The results demonstrate the potential for creating the equivalent of full-dose, contrast-enhanced MR images without any contrast agent use.
- RSNA Media Relations
1-630-590-7762
media@rsna.org - RSNA 2018 Newsroom
(Nov. 24-29, 2018)
1-312-791-6610 - Maureen Morley
1-630-590-7754
mmorley@rsna.org - Linda Brooks
1-630-590-7738
lbrooks@rsna.org
CHICAGO – Researchers are using artificial intelligence to reduce the dose of a contrast agent that may be left behind in the body after MRI exams, according to a study being presented today at the annual meeting of the Radiological Society of North America (RSNA).
Gadolinium is a heavy metal used in contrast material that enhances images on MRI. Recent studies have found that trace amounts of the metal remain in the bodies of people who have undergone exams with certain types of gadolinium. The effects of this deposition are not known, but radiologists are working proactively to optimize patient safety while preserving the important information that gadolinium-enhanced MRI scans provide.
“There is concrete evidence that gadolinium deposits in the brain and body,” said study lead author Enhao Gong, Ph.D., researcher at Stanford University in Stanford, Calif. “While the implications of this are unclear, mitigating potential patient risks while maximizing the clinical value of the MRI exams is imperative.”
Dr. Gong and colleagues at Stanford have been studying deep learning as a way to achieve this goal. Deep learning is a sophisticated artificial intelligence technique that teaches computers by examples. Through use of models called convolutional neural networks, the computer can not only recognize images but also find subtle distinctions among the imaging data that a human observer might not be capable of discerning.
To train the deep learning algorithm, the researchers used MR images from 200 patients who had received contrast-enhanced MRI exams for a variety of indications. They collected three sets of images for each patient: pre-contrast scans, done prior to contrast administration and referred to as the zero-dose scans; low-dose scans, acquired after 10 percent of the standard gadolinium dose administration; and full-dose scans, acquired after 100 percent dose administration.
The algorithm learned to approximate the full-dose scans from the zero-dose and low-dose images. Neuroradiologists then evaluated the images for contrast enhancement and overall quality.
Results showed that the image quality was not significantly different between the low-dose, algorithm-enhanced MR images and the full-dose, contrast-enhanced MR images. The initial results also demonstrated the potential for creating the equivalent of full-dose, contrast-enhanced MR images without any contrast agent use.
These findings suggest the method’s potential for dramatically reducing gadolinium dose without sacrificing diagnostic quality, according to Dr. Gong.
“Low-dose gadolinium images yield significant untapped clinically useful information that is accessible now by using deep learning and AI,” he said.
Now that the researchers have shown that the method is technically possible, they want to study it further in the clinical setting, where Dr. Gong believes it will ultimately find a home.
Future research will include evaluation of the algorithm across a broader range of MRI scanners and with different types of contrast agents.
“We’re not trying to replace existing imaging technology,” Dr. Gong said. “We’re trying to improve it and generate more value from the existing information while looking out for the safety of our patients.”
Co-authors are Jonathan Tamir, B.Sc., John Pauly, Max Wintermark, M.D., and Greg Zaharchuk, M.D., Ph.D.
Dr. Gong received an RSNA “Trainee Research Prize – Resident” award for his research.
Note: Copies of RSNA 2018 news releases and electronic images will be available online at RSNA.org/press18 beginning Monday, Nov. 26.
RSNA is an association of over 54,000 radiologists, radiation oncologists, medical physicists and related scientists, promoting excellence in patient care and health care delivery through education, research and technologic innovation. The Society is based in Oak Brook, Ill. (RSNA.org)
Editor's note: The data in these releases may differ from those in the published abstract and those actually presented at the meeting, as researchers continue to update their data right up until the meeting. To ensure you are using the most up-to-date information, please call the RSNA Newsroom at 1-312-791-6610.
For patient-friendly information on contrast materials, visit RadiologyInfo.org.
Images (JPG, TIF):
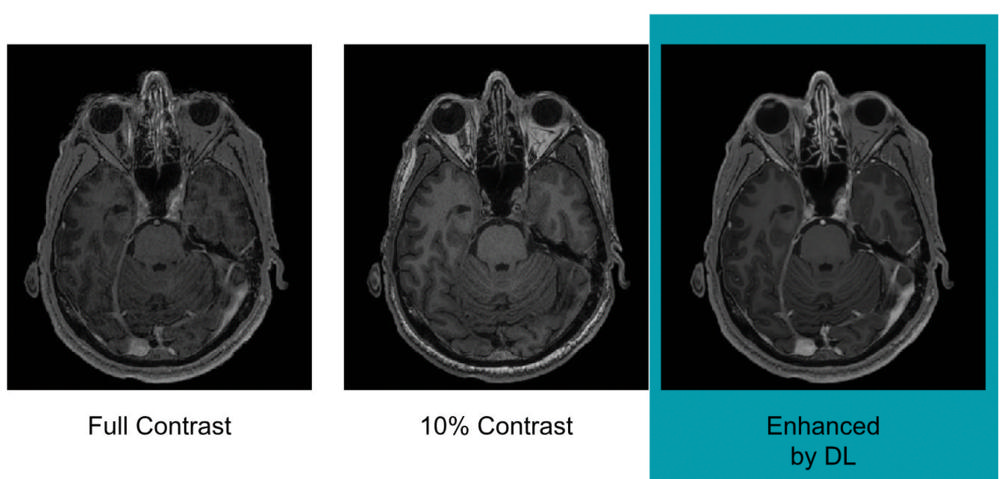
Figure 1. Example of full-dose, 10 percent low-dose and algorithm-enhanced low-dose.
High-res (TIF) version
(Right-click and Save As)
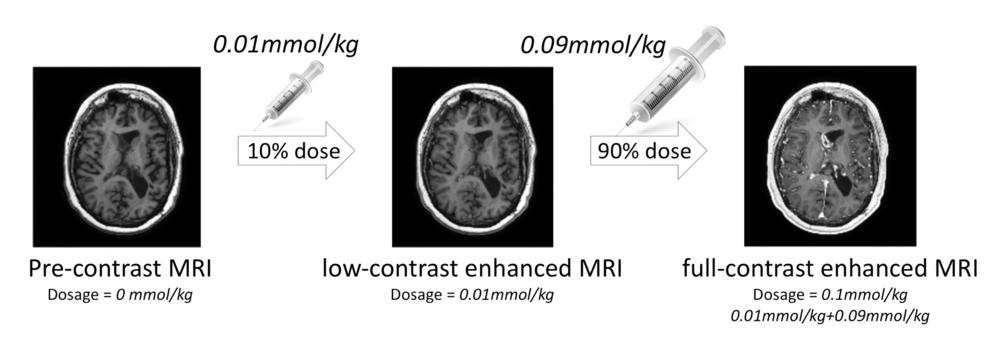
Figure 2. Imaging protocol used with 3 different MR series at different contrast doses.
High-res (TIF) version
(Right-click and Save As)
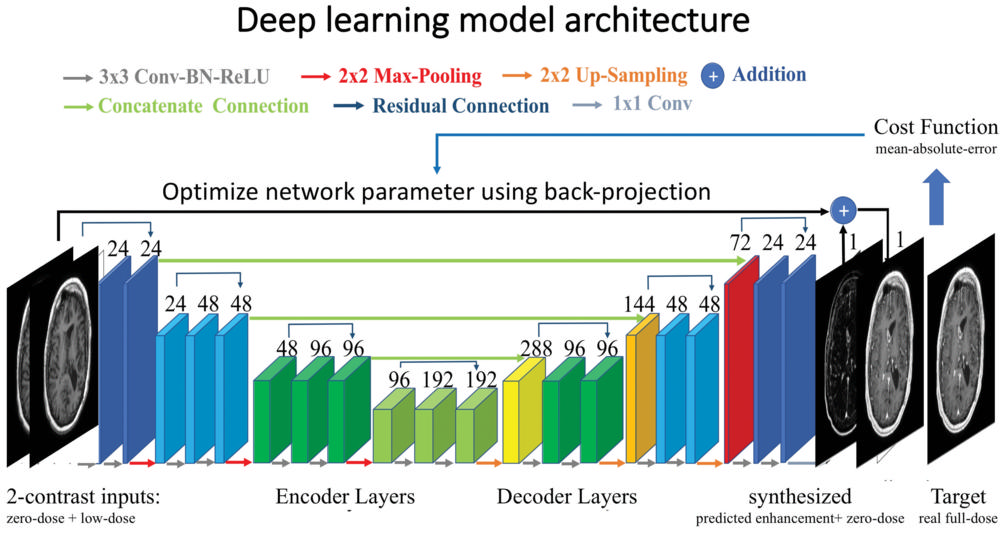
Figure 3. Visualization of the deep learning model architecture that was used.
High-res (TIF) version
(Right-click and Save As)
Video (MP4):
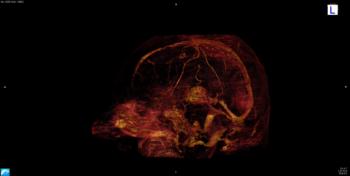
Video 1. Video shows volumetric visualization of algorithm-enhanced 10 percent contrast information.
Download MP4
(Right-click and Save As)
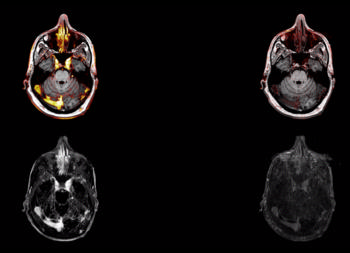
Video 2. Video shows comparison of enhanced contrast vs 10 percent contrast on a tumor case.
Download MP4
(Right-click and Save As)
Information for Consumers: