AI Improves Efficiency and Accuracy of Digital Breast Tomosynthesis
Released: July 31, 2019
At A Glance
- Researchers trained an AI system on large digital breast tomosynthesis (DBT) data sets to identify suspicious findings.
- The system was tested by having 24 radiologists, including 13 breast subspecialists, each read 260 DBT examinations with and without AI assistance.
- Cancer detection sensitivity and specificity increased, and reading times decreased.
- RSNA Media Relations
1-630-590-7762
media@rsna.org - Linda Brooks
1-630-590-7738
lbrooks@rsna.org - Dionna Arnold
1-630-590-7791
darnold@rsna.org
OAK BROOK, Ill. — Artificial intelligence (AI) helps improve the efficiency and accuracy of an advanced imaging technology used to screen for breast cancer, according to a new study published in the journal Radiology: Artificial Intelligence.
Digital breast tomosynthesis (DBT) is an advanced method for cancer detection in which an X-ray arm sweeps over the breast, taking multiple images in a matter of seconds.
Research has shown that DBT improves cancer detection and reduces false-positive recalls compared to screening with digital mammography (DM) alone. However, the DBT exam can take almost twice as long to interpret as DM due to the time it takes for the radiologist to scroll through all the images. This increased time is likely to be more consequential as DBT increasingly becomes the standard-of-care for mammographic imaging.
For the study, researchers developed a deep learning system, a type of AI that can mine vast amounts of data to find subtle patterns beyond human recognition. They trained the AI system on large DBT data sets to identify suspicious findings in the DBT images.
After developing and training the system, the researchers tested its performance by having 24 radiologists, including 13 breast subspecialists, each read 260 DBT examinations with and without AI assistance. The examinations included 65 cancer cases.
Use of AI was associated with improved accuracy and shorter reading times. Sensitivity increased from 77 percent without AI to 85 percent with it. Specificity increased from 62.7 percent without AI to 69.6 percent with it. The recall rate for non-cancers, or the rate at which women were called back for follow-up examinations based on benign findings, decreased from 38 percent without AI to just 30.9 percent with it. On average, reading time decreased from just over 64 seconds without AI to only 30.4 seconds with it.
“Overall, readers were able to increase their sensitivity by 8 percent, lower their recall rate by 7 percent and cut their reading time in half when using AI concurrently while reading DBT cases compared to reading without using AI,” said study lead author Emily F. Conant, M.D., professor and chief of breast imaging from the Department of Radiology at the Perelman School of Medicine at the University of Pennsylvania in Philadelphia.
Also showing improvement was the area under the receiver operating characteristic curve (AUC), a graphing variable that combines sensitivity and specificity into a single measure for a better representation of overall radiologist performance. Radiologist performance, measured by mean AUC, increased from 0.795 without AI to 0.852 with AI.
“We know that DBT imaging increases cancer detection and lowers recall rate when added to 2-D mammography and even further improvement in these key metrics is clinically very important,” Dr. Conant said. “And, since adding DBT to the 2-D mammogram approximately doubles radiologist reading time, the concurrent use of AI with DBT increases cancer detection and may bring reading times back to about the time it takes to read DM-alone exams.”
The researchers expect the deep learning approach to improve as it is exposed to larger and larger data sets, making its potential impact on patient care even more significant.
“The results of this study suggest that both improved efficiency and accuracy could be achieved in clinical practice using an effective AI system,” Dr. Conant said.
“Improving Accuracy and Efficiency with Concurrent Use of Artificial Intelligence for Digital Breast Tomosynthesis.” Collaborating with Dr. Conant were Alicia Y. Toledano, Sc.D., Senthil Periaswamy, Ph.D., Sergei V. Fotin, Ph.D., Jonathan Go, M.A.Sc., Justin E. Boatsman, M.D., and Jeffrey W. Hoffmeister, M.D., MSEE.
Radiology: Artificial Intelligence is edited by Charles E. Kahn Jr., M.D., University of Pennsylvania (Penn) Perelman School of Medicine, Philadelphia, and owned and published by the Radiological Society of North America, Inc. (https://pubs.rsna.org/journal/ai)
RSNA is an association of over 53,400 radiologists, radiation oncologists, medical physicists and related scientists promoting excellence in patient care and health care delivery through education, research and technologic innovation. The Society is based in Oak Brook, Ill. (RSNA.org)
For patient-friendly information on breast tomosynthesisRadiologyInfo.org.
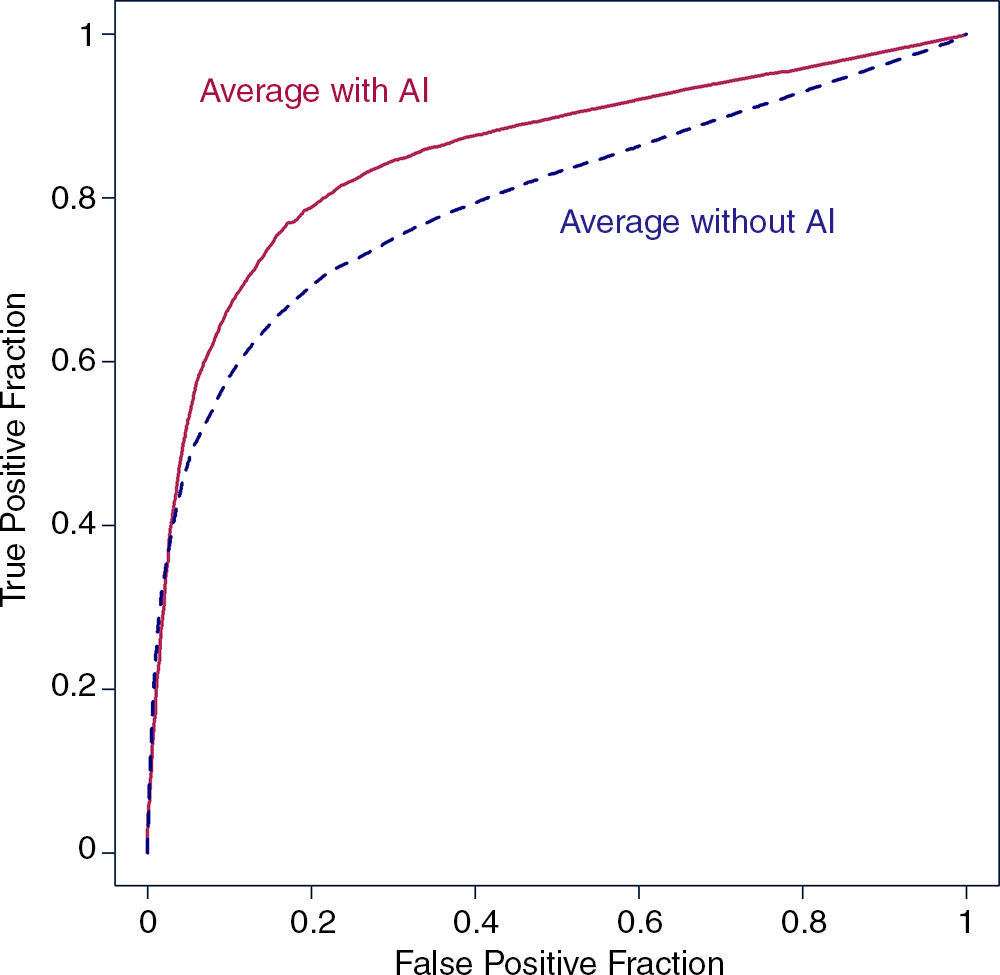
Figure 2. Average of empirical receiver operating characteristic plots with and without artificial intelligence (AI). True-positive fraction = case-level sensitivity, false-positive fraction = 1 − specificity.
High-res (TIF) version
(Right-click and Save As)
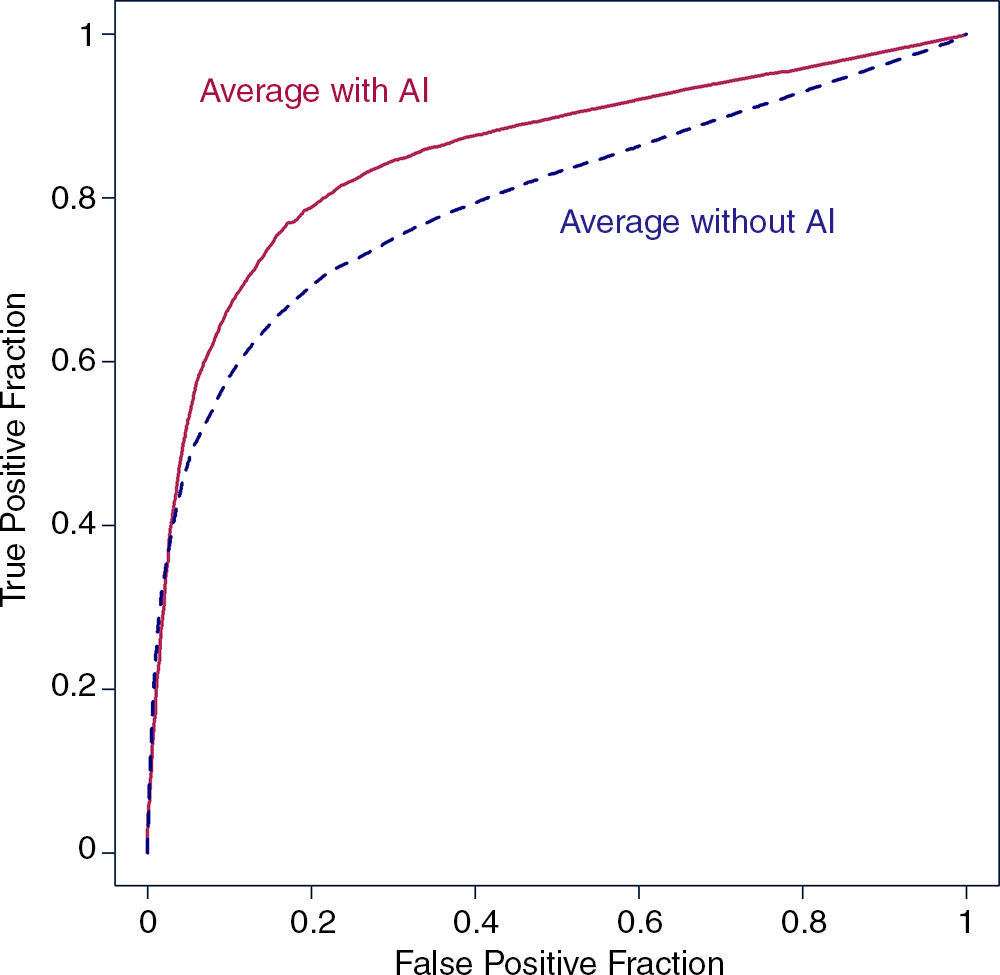
Figure 3. Bar graph shows average reading times for each reader without and with artificial intelligence (AI).
High-res (TIF) version
(Right-click and Save As)
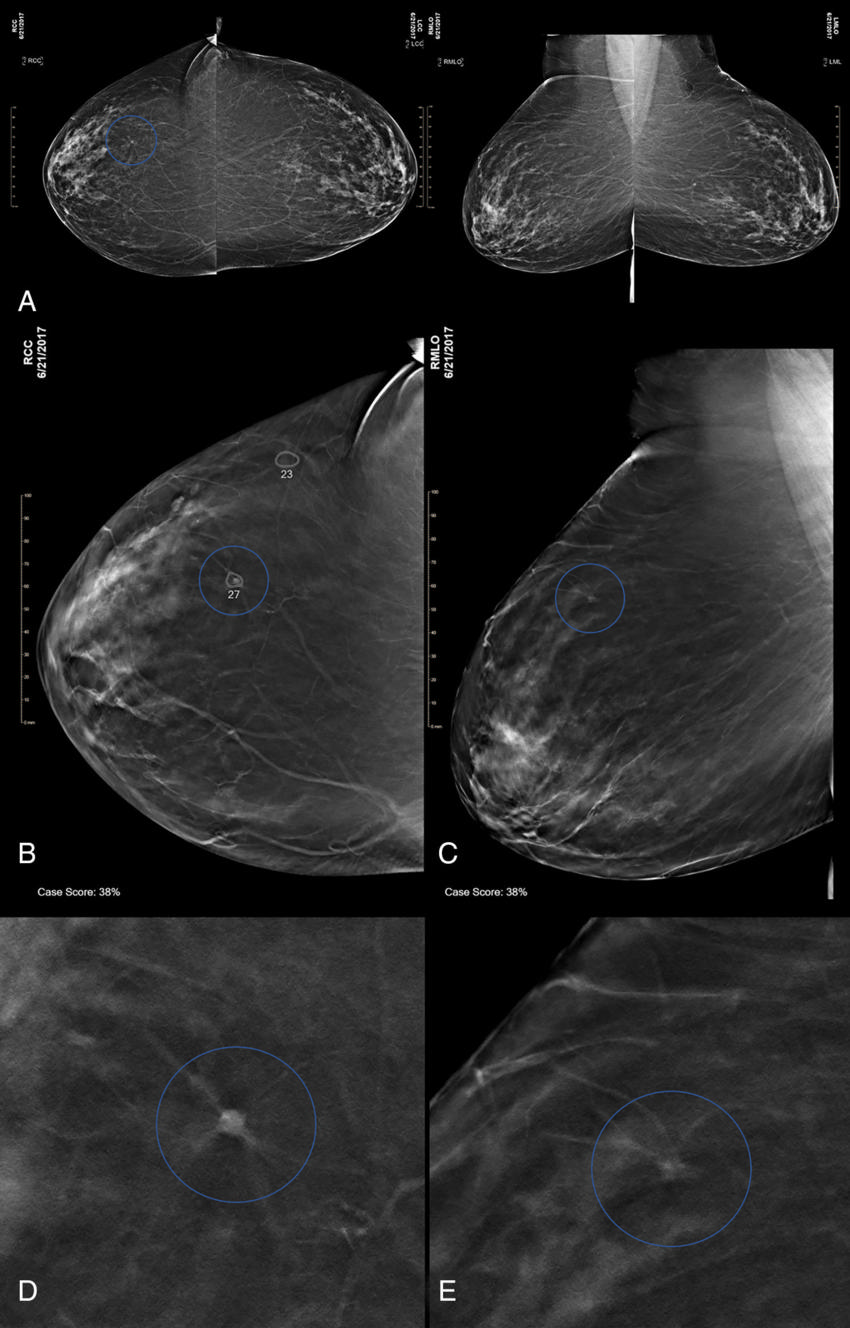
Figure 4. Images in a 74-yearold woman at screening with combination digital mammography (DM) and digital breast tomosynthesis (DBT).
High-res (TIF) version
(Right-click and Save As)
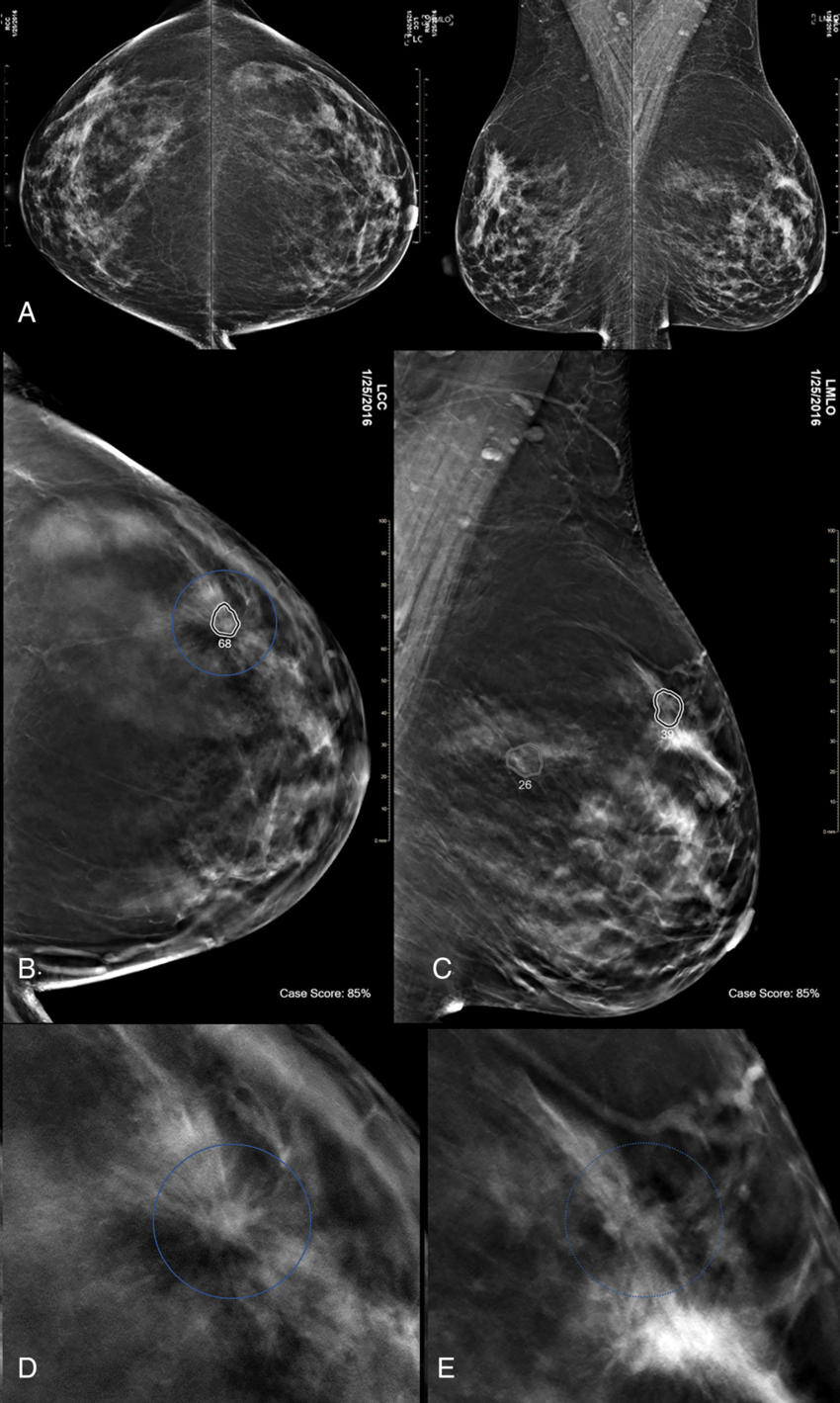
Figure 5. Images in a 47-yearold woman at screening with combination digital mammography (DM) and digital breast tomosynthesis (DBT).
High-res (TIF) version
(Right-click and Save As)
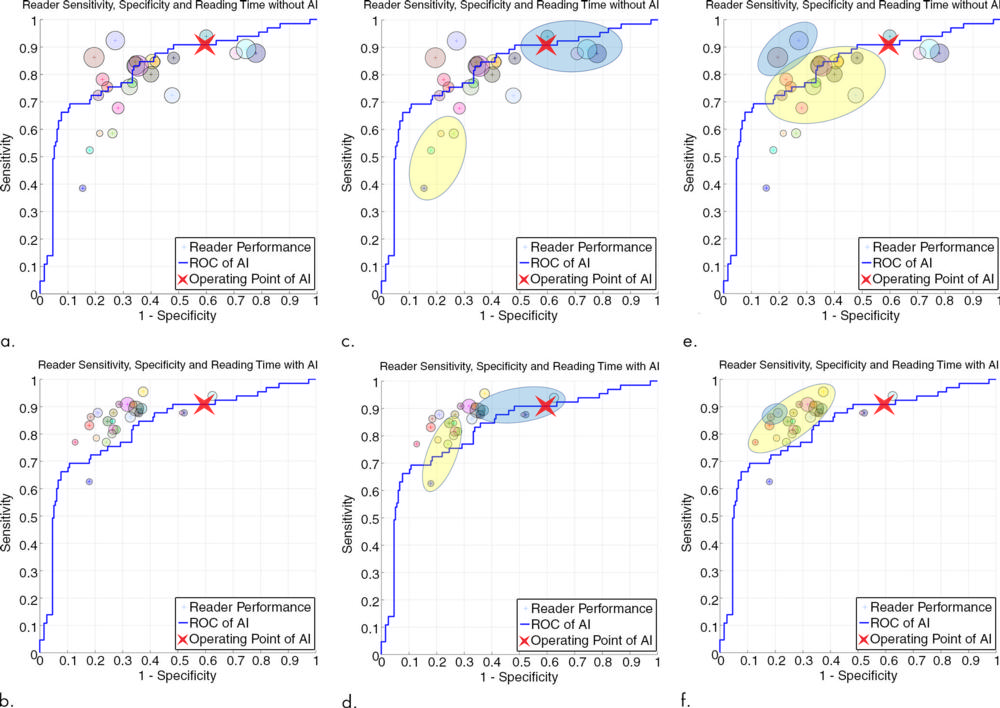
Figure 6. Graphs show (a, c, e) average case-level performance for each reader without artificial intelligence (AI) and (b, d, f) with AI. Locations of small circles = sensitivity and specificity. Diameters of small circles are proportional to reading time; a decrease in circle size from readings without AI to readings with AI reflects the relative decrease in reading time for the individual reader. (c–f) Graphs highlight specific groups of readers and their changes in sensitivity, specificity, and reading time. The large yellow circle in c and d shows a group of four readers who without AI have high specificity, low sensitivity, and short reading times. With AI, these readers maintain their relatively short reading times and high specificity but improve their sensitivity. As demonstrated by the large blue circle in c and d, four readers who have low specificity but high sensitivity without AI improve their reading times and specificity and maintain their relatively high sensitivity. The large yellow circle in e and f shows a group of readers with generally high sensitivities and specificities without AI who with AI generally improve all three parameters (reading time, sensitivity, and specificity). The large blue circle in e and f indicates the only two readers in our study who had slight decreases in area under the receiver operating characteristic (ROC) curve (AUC) when reading with AI compared with reading without AI. Their reductions in AUC were quite small at −0.014 (right small circle) and −0.004 (left small circle); however, they both experienced significant reductions in reading time. The right reader had the fourth largest reduction of all readers of −65.7 seconds, and the left reader had the largest reduction of all, −90.4 seconds. (a–f) Graphs also show the AI stand-alone performance ROC curve (no human reader, blue line) and operating point (red “X”) with the 260 enriched reader study cases. The AI operating point case-level sensitivity was 91 percent (59 of 65; 95 percent confidence interval [CI]: 81 percent, 96 percent), and its specificity was 41 percent (79 of 195; 95 percent CI: 34 percent, 48 percent).
High-res (TIF) version
(Right-click and Save As)